Key Takeaways
- An autonomous system can achieve a specific set of goals in a changing environment by gathering information about the environment without human intervention!
- An autonomous system can achieve a specific set of goals in a changing environment by gathering information about the environment without human intervention!
- GenAI has introduced an agent-based approach to integrate technology into day-to-day operations and generate value. The agent-based approach offers a repeatable framework and process to embed GenAI into their workflows.
- Autonomous operations start with the automation of routine processes through GenAI agents. The next stage is automation of workflows followed by enterprise-level automation and finally leading to an autonomous business.
- GenAI agents not only enhance the existing processes but also pave the way for growth and competitive advantage.
In this day and age of AI (Artificial Intelligence), we are all witness to how systems, operations, processes, etc., have undergone a sea of change. From manual systems, we progressed to partially automated systems and then to fully automated systems. However, all these automated systems require human intervention!
Further advancements in AI have opened doors to a plethora of opportunities for creating innovative products that make our lives easy and boost business efficiency.
The latest offering is autonomous systems! A system that can achieve a specific set of goals in a changing environment by gathering information about the environment without human intervention!
A classic example of an autonomous system that we are seeing is self-driving cars.
- How are Autonomous Systems Different from Automated Systems?
- How GenAI Drives Autonomous Operations?
- How to Build an Autonomous Platform?
- How Autonomous Systems Help you to Scale your Business?
- Tips to Build an Autonomous Business
- Challenges in Implementing Autonomous Systems
- A Peek into the Autonomous Future
- Conclusion
An autonomous system can keep track of the current state of the system and its location. It perceives and understands disparate data sources. It can make a plan and determine what action is required to be taken in any situation. Autonomous systems are designed such that they act only when it is safe for them to do so ensuring that they avoid risk to human safety, property, or the autonomous system.
Autonomous systems are often confused with automated systems. However, they are quite different from each other!
How are Autonomous Systems Different from Automated Systems?
An automated system, powered by RPA (Robotic Process Automation), runs within a well-defined set of parameters. The decisions they make and the actions they take are based on how the algorithms are coded. Hence, they are limited in the types of tasks they can perform.
Autonomous systems in contrast learn and adapt to dynamic environments. These systems evolve as their environment changes. Autonomous systems learn and adapt to conditions way beyond what they had initially been programmed for. These systems are capable of learning from increasing datasets at a much faster pace with reliability when compared to a human.
An automated system that was designed with a specific set of inputs and outputs can be made “smart” by integrating technology. Hence, automated systems can become autonomous thereby extending the useful life of the system and its applicability.
Autonomous systems embody a shift towards the future where bots will not only perform tasks but also think, learn, and make decisions independently. At the core of autonomous systems are technologies like AI (Artificial Intelligence), ML (Machine Learning), and Generative AI.
Powered by these technologies autonomous systems ingest and analyze vast volumes of data, learn from patterns and anomalies, and optimize operations independently.
How GenAI Drives Autonomous Operations?
GenAI has introduced an agent-based approach to integrate technology into their day-to-day operations and generate value. The agent-based approach offers a repeatable framework and process to embed GenAI into their workflows.
Agents not only perform the tasks that they have been assigned but also have decision-making capabilities making them truly autonomous.
GenAI agents are powered by enhanced computational power, breakthroughs in ML, and the availability of vast volumes and a variety of data.
GenAI agents leverage their training data and knowledge that they can access and contextually inform their decisions and responses. They use real-time data and interact with other systems. Agents also keep track of completed tasks, user input, etc.
GenAI agents collaborate with other agents to share insights and coordinate efforts to achieve collective goals.
Agents can be deployed across the organization in departments like human resources, marketing, engineering, etc. Integrating GenAI agents into various operational areas makes a shift beyond increasing operational efficiency.
Because of the promise GenAI holds, it is being widely adopted worldwide. According to Bloomberg International, Generative AI is likely to become a $1.3 trillion market by 2032. (Source: Exploding Topics)
How to Build an Autonomous Platform?
- Establish your Automation Goals
It is essential to establish what you expect to achieve with automation. A clear goal will help you align all your resources and activities for achieving it,
- Integrate Relevant Data Sources
High-quality, relevant data is a critical element for GenAi-based systems to function. Therefore, you will need to identify the relevant data sources and integrate them with the platform to facilitate real-time decision-making.
- Leverage ML (Machine Learning)
ML algorithms analyze data, make forecasts, and identify patterns, anomalies, and opportunities for optimization. All these data points power GenAI models to make decisions in different scenarios.
- Automate Processes
Automate processes and workflows to streamline operations, minimize manual tasks, and enhance operational efficiency, Automation reduces errors and boosts the speed of processes.
- Track Performance
Monitor the performance of your autonomous operations. Train the ML algorithms and refine workflows to ensure that the model adapts to evolve according to the changing business environment.
How Autonomous Systems can be Scaled?
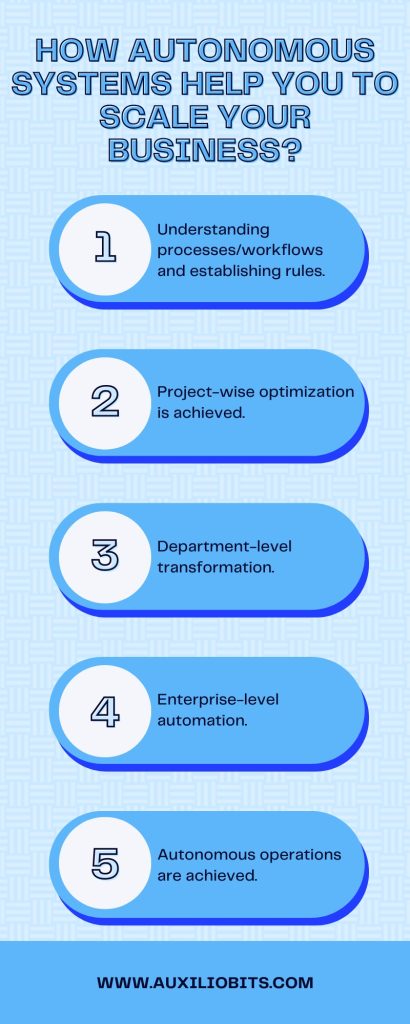
Autonomous systems go through five stages of maturity as they evolve from manual processes to autonomous operations. During each of these stages, technology improves over time and it can accomplish more in less time.
The five stages are:
- Step 1
This stage includes all activities that focus on understanding processes/workflows and establishing rules.
At this stage, GenAI is used to document processes and workflows.
Processes are turned into standard operating procedures (SOPs) with the help of GenAI tools like ChatGPT.
GenAI tools are used to create templates, checklists, and other operational documents that save time.
- Step 2
After the foundation is laid in the previous step, project-wise optimization is achieved in stage 2.
GenAI tools analyze project-specific data gathered from disparate sources and provide insights to build project roadmaps.
The enterprise data is augmented by passing data or integrating search engines to locate and extract any information that helps to construct a prompt.
LLMs (Large Language Models) are trained to complete different tasks in the projects.
Self-contained processes are automated first. The automation is enhanced with rapid iterations based on user feedback.
Repetitive tasks that can be automated are documented.
- Step 3
After project-level automation, the focus shifts to department-level transformation.
GenAI tools are provided access to current proprietary and contextual data.
“Chain-of-thought” prompts are created by different departments. These prompts help LLMs to fragment intricate problems into smaller sequential steps.
The workflows in each department are automated to boost efficiency.
These workflows are integrated into other systems with the help of task-specific enterprise assistants like CRMs, etc., and plug-ins.
GenAI is leveraged for making department-level forecasts that facilitate future planning.
Customer service bots that can answer contextual questions are created by leveraging GenAI.
- Step 4
Once department-level automation is achieved, the stage is set for enterprise-level automation which requires seamless integration of departments.
AI automates collaboration between departments and seamless exchange of data thereby facilitating cross-department data-driven decision making.
Autonomous agents are built using LLMs and other tools. These agents are deployed to complete multi-step tasks and automate workflows that do not follow predetermined rules. Agents access LLMs recursively and check for enterprise data and tools that are aligned with the context and previous interactions.
Data taxonomy and knowledge graphs are standardized across the organization. A centralized repository is developed to store all the initial information from all enterprise systems.
LLMs are modeled with enterprise data so that they can perform new tasks and respond to new patterns.
GenAI tools optimize predictions and provide insights to an AI assistant advisor who tracks decisions against goals and recommends enhancements.
- Step 5
Autonomous operations are achieved
AI and humans work together. GenAI models provide predictive models and simulations that can be accessed by team members through user-friendly interfaces.
Autonomous operations are achieved in part following which workflows are automated and optimized.
Multiple agents operate cohesively to solve complex problems. Jobs are broken down into modular steps and assigned to each agent. An organization structure with autonomous agents is built where each agent is assigned a specific task. Building a hierarchal structure and defining roles prevents unproductive loop hallucination.
The number of agents needs to be increased to scale the system.
GenAI agents execute several complex processes in tandem whilst continually improving themselves through reinforcement learning and enhancing processes automatically.
All the above steps lead to a scalable autonomous enterprise in which both people and systems are continually Improving.
Tips to Build an Autonomous Business
An autonomous business is partially governed by software agents that are also responsible for operating it. Autonomous business integrates smart products, machine customers, and a programmable economy.
While deploying GenAI will offer a competitive advantage, businesses must be aware that once the adoption of the technology becomes commonplace, they will lose that advantage. Hence, businesses must not just rely on technology but focus on enhancing internal AI skills, infrastructure, and workflows for a sustainable future.
The business must define policies, procedures, practices, and principles within which the autonomous system functions.
The most effective strategy to build an autonomous business is to create different autonomous agents and assign them specific roles. These agents must have clearly defined roles in a virtual organizational chart that mimics real-time team dynamics.
The autonomous agents must be trained to work cohesively within the limitations of the system to enhance productivity.
Although autonomous agents function without human intervention, you must establish governance over the decision-making abilities of agents. Must ensure that roles, SOPs, decision-making, and process responsibilities function as planned.
However, the implementation of autonomous systems has its set of challenges.
Challenges in Implementing Autonomous Systems
- While attempting to integrate GenAI with your existing systems, you might face problems like compatibility issues, data migration challenges, etc.
- You might face a shortage of skilled professionals.
- Some crucial factors while implementing GenAI are data privacy concerns, ensuring ethical use of algorithms, compliance with regulations, etc.
- Another factor that makes it difficult to implement GenAI in organizations is resistance to change. Therefore, due attention must be given to drive a cultural transformation across the organization.
- It is equally important to build trust with customers and stakeholders to drive innovation, sustainability, and efficiency with GenAI.
A Peek into the Autonomous Future
Technology is evolving at a breakneck pace and Generative AI has opened doors to unlimited possibilities. The future with GenAI looks promising!
We are likely to see autonomous agents within departments that will be integrated into each other across the enterprise paving the way for an autonomous enterprise that functions with minimal human intervention.
GenAI models will increase their capabilities through reinforced learning from human feedback.
We can also expect domain-specific GenAI models with intelligent decision-making abilities.
Conclusion
GenAI agents promise a future where incremental enhancements lead to significant operational transformation. These agents help organizations stay at the forefront of digital innovation. They not only enhance the existing processes but also pave the way for growth and competitive advantage.
Autonomous business is the future! And, if you don’t want to be left behind, it is time to ramp up your business with digital transformation! The team at Auxiliobits is there to make your automation journey. Our domain-specific experts understand the needs of your business and suggest strategies accordingly. We provide cost-effective digital solutions that are tailored according to the unique needs of your business.