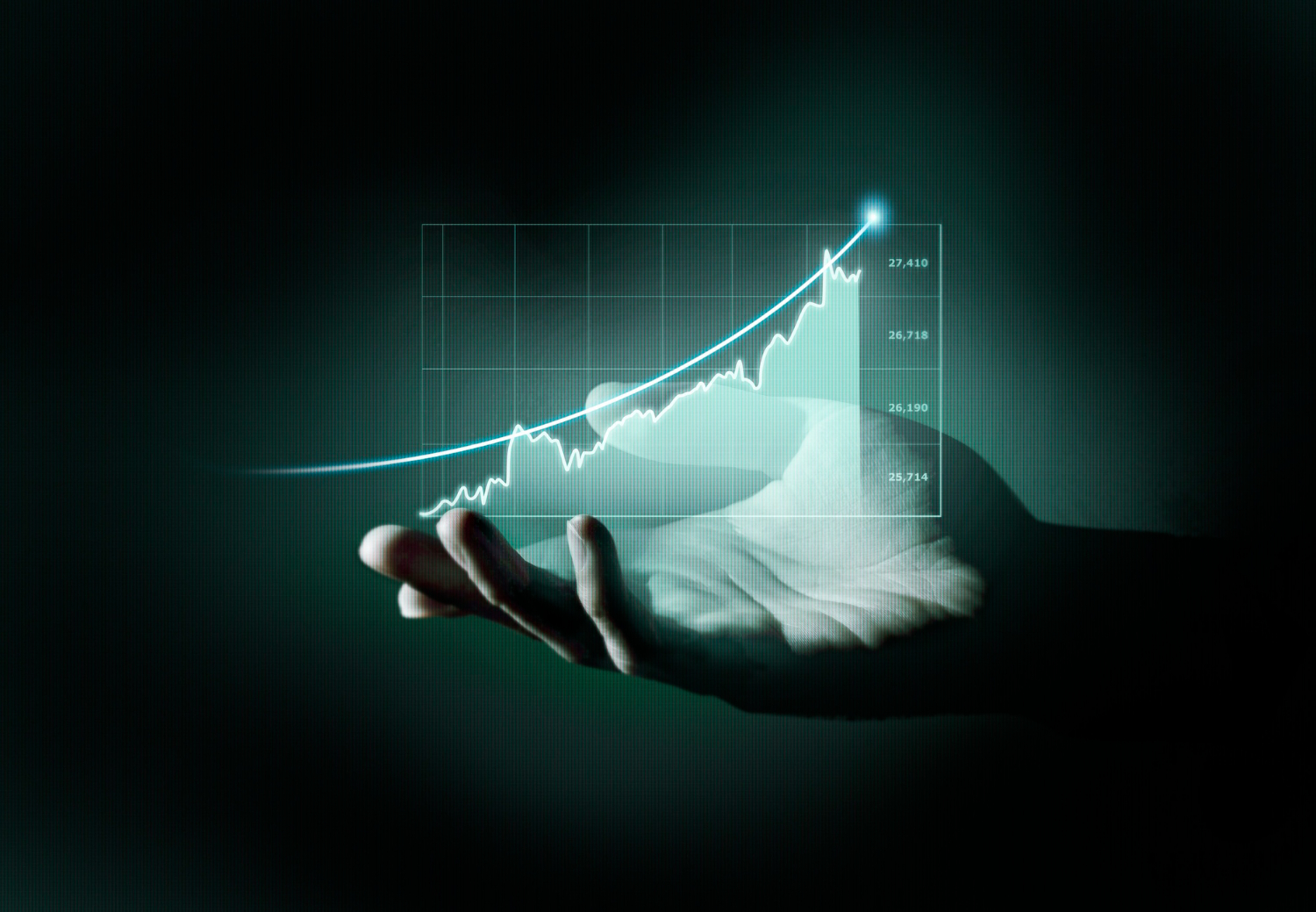
Key takeaways
- The financial sector needs to respond to factors like high-interest rates, regulatory compliance, inflation, and more. In addition to this, they need to adapt to disruptive technologies.
- GenAI models are deployed across sectors for functions like content creation, GenAI-powered virtual assistants, creating innovative designs, data analysis, code generation, and more. GenAI boosts productivity and enhances outcomes.
- GenAI models generate synthetic and informative financial scenarios that help enhance portfolio optimization strategies, facilitate sophisticated risk simulations, fraud detection, and more.
- Generative AI is being deployed to enhance outcomes in financial services like fraud detection and prevention, virtual assistants, personalized products, and more.
- The generative AI technology has the power to revolutionize financial services and enhance outcomes provided it is deployed appropriately.
- Generative AI is reinventing finance by automating processes, creating synthetic data, and providing valuable insights for making strategic decisions.
The financial services industry has been a strong force in helping people and businesses cope with societal and economic changes. This sector responds to changing conditions and facilitates business transformations.
In recent years, the financial sector has had to respond to factors like high-interest rates, regulatory compliance, inflation, and more. In addition to this, they need to adapt to disruptive technologies.
Automation technologies like RPA (Robotic Process Automation), AI (Artificial Intelligence), etc. have made huge impacts in the financial sector. From streamlining processes to boosting efficiencies, AI technologies have proved to have been game changers in the financial domain!
In 2024, the financial services sector will need to look into tapping technologies like Generative AI, transition to the cloud, increased cyber and fraud risks, and more.
Generative AI is one technology that promises to have revolutionary impacts in the financial sector.
Generative AI: An Overview
GenAI is a technology that made an emphatic presence in 2022 and has continued to remain a strong force to reckon with ever since!
Generative AI is a discipline of AI that leverages advanced algorithms and neural networks to create new content in the form of text, image, video, audio, etc., that resembles the content created by humans.
Generative AI deploys advanced ML (Machine Learning) models that are trained using large datasets. These models can identify patterns, correlations, structure, etc., within datasets and generate new content based on it. Although the new data resembles the training data, it is different.
GenAI models are being deployed across sectors for functions like content creation, GenAI-powered virtual assistants, creating innovative designs, data analysis, code generation, and more. GenAI boosts productivity and enhances outcomes.
According to Bloomberg International, the Gen AI market is expected to reach a whopping $ 1.3 trillion by 2032. (Source: https://explodingtopics.com/blog/generative-ai-stats)
Generative AI is relevant to the financial services industry because it overcomes the shortcomings of real-world data and facilitates personalized customer experiences, improved risk assessments, fraud detection, and smart investment management.
Generative AI and Finance
The enhancements provided by GenAI have made generative AI tools extremely popular among financial services professionals. GenAI is being integrated into financial operations to drive innovation and gain a competitive advantage.
GenAI creates synthetic data that closely resembles real-world financial data. This synthetic data is used for training ML algorithms boosting their capability to identify patterns, detect trends, and make accurate predictions. GenAI models overcome the limitations of real-world data like missing data, biased samples, etc.
GenAI tools automate manual processes like data analysis, fraud detection, and more. Automation enhances operational efficiency and speed while reducing human errors. Integration of GenAI in financial processes also reduces costs.
Generative AI models analyze vast volumes of financial data, trading volumes, and market indicators and provide insights to finance professionals. These insights help finance professionals make data-driven investment decisions, and build risk management strategies, and fraud detection methods.
The integration of generative AI in finance includes the potential to redefine traditional approaches. GenAI models generate synthetic and informative financial scenarios that help enhance portfolio optimization strategies, facilitate sophisticated risk simulations, fraud detection, and more.
Hence, it comes as no surprise that the global market size for GenAI in financial services is expected to reach $ 9475.2 million by 2032 recording a CAGR of 28.1% for a forecast period from 2022 to 2032. (Source: Leeway Hertz)
Use Cases of Gen AI in Finance
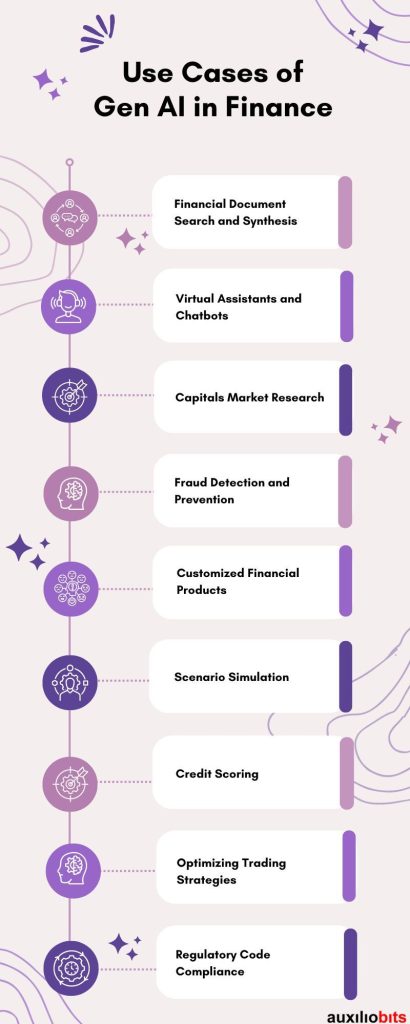
- Financial Document Search and Synthesis
GenAI models analyze large sets of specialized documents and can help finance professionals comprehend the information in various documents such as contracts, unstructured PDF documents, etc.
Generative AI tools can also research and summarize different economic data and statistics across the globe. It also helps financial services by creating comprehensive and intuitive pitch books and presentation materials.
- Virtual Assistants and Chatbots
GenAI-powered chatbots engage with users just like humans would. These bots can comprehend context, engage in multidimensional dialogue, retain recollections, and more to offer customers a great experience.
Chatbots leverage NLP models to extract relevant data such as intent classification, entity recognition, and sentiment analysis from user inputs. Hence, these chatbots can comprehend user requests and provide appropriate responses accurately in a quick time.
Generative AI chatbots analyze vast volumes of customer data and offer customers personalized guidance on aspects like spending, savings, and investments. These bots can also alert users in case of anomalies to prevent fraud.
Besides offering these services to customers, GenAI bots relieve the burden of customer service agents as well.
- Capitals Market Research
GenAI models work as research assistants for investment analysis in the capital market. These models scan through billions of event transcripts, company filings, macroeconomic reports, and other relevant resources. They identify and summarize vital information from this data and provide it to finance teams at a high speed.
- Fraud Detection and Prevention
Generative AI agents leverage adaptive deep learning models to provide a multitude of fraud detection possibilities. These models analyze transactional behavior, spending patterns, risk indicators, and customer data to detect anomalies. They flag anomalies associated with fraud in real time so that timely action can be taken to prevent fraud.
GenAI models analyze the historical payment behavior of customers and predict credit default risks facilitating proactive risk management.
Generative AI algorithms conduct in-depth risk evaluations based on historical data, present patterns, and probable changes to create predictive models. These predictive models along with situation analysis help estimate the probability and impact of recognized threats. It thereby facilitates the formulation of data-based risk reduction strategies.
One of the best aspects of generative AI in fraud detection is that these models learn and adapt continuously. Therefore, as new fraudulent activities emerge GenAI models align their detection methods without requiring human intervention.
- Customized Financial Products
Generative AI models analyze vast datasets and intricate market trends to get a better understanding of the unique needs of each customer. These models can assess the risk tolerance, financial profile, and long-term goals of each customer. Based on this data, GenAI models can create personalized investment strategies, insurance plans, etc. Personalized financial products help enhance customer experience.
- Scenario Simulation
GenAI models simulate real-world scenarios based on multiple factors and variables. Based on these simulations, finance professionals can anticipate potential outcomes and assess the robustness of their strategies. GenAI helps finance service providers make better data-driven decisions.
- Credit Scoring
GenAI models provide finance professionals with a holistic view of consumer behavior and credit history. These models augment existing data by generating synthetic data that resembles the existing data. The synthetic data can be used for training robust models for credit scoring. Generative AI also helps assess the creditworthiness of borrowers accurately by identifying non-traditional variables or patterns. These insights help finance service providers make data-based lending decisions.
- Optimizing Trading Strategies
Generative AI models correlate data from multiple sources and process them in real time to identify sentiments that can impact trades. This analysis helps finance professionals develop robust trading strategies.
GenAI models learn from past experiences and dynamically alter their strategies in real-time thereby facilitating a more efficient and adaptive approach to trading decision-making.
- Regulatory Code Compliance
One of the biggest challenges for the financial sector is keeping pace with regulatory terms and ensuring compliance. GenAI models provide developers the context about the regulatory/business change that will require modifications by providing them summarized answers. GenAI models streamline code change by automating the process and providing documentation.
The generative AI technology has the power to revolutionize financial services and enhance outcomes provided it is deployed appropriately. Otherwise, it can lead to complications.
How to Implement Gen AI in Finance?
- Establish your automation goals
Identify the business objectives and challenges that GenAI can address. You will also need to identify the specific use cases where GenAI tools can provide value.
- Ensure that your Business is Ready
The deployment of generative AI models for your financial service business will require a change in mindset. You will need to ensure that your leadership and team members are ready to accept the changes that will be brought about by implementing automation.
Since GenAI bots will take over several tasks from humans, you will need to make necessary changes in your workforce, roles, and responsibilities so that your business is aligned with the demands of technology.
- Integrate Data
Data quality and integration are of extreme importance because GenAI models depend on data to function. Make sure that you provide high-quality data for training the models. It is also important to integrate multiple sources of data such as transaction records, customer data, etc. so that it can be accessed easily to train GenAI models.
- Choose ML Models
The choice of ML model will depend on the application. For instance, document processing will require a different software compared to chatbots which will require Natural Language Processing (NLP) models. Therefore, make sure that you choose the ML model that is best suited for your specific use case.
- Train ML Models
The next step is training the models so that they can perform the desired function. ML models can be trained for specific use cases like fraud detection, virtual assistance, regulatory compliance, and more!
ML models are trained using historical data relevant to the use case. It is equally important to update the models continuously so that the model can align with changing patterns and trends.
- Automate Processes
Leverage RPA (Robotic Process Automation) to automate rule-based processes like data entry, document processing, etc. Automation of routine processes streamlines workflows and boosts efficiency while also reducing costs.
- Measure Output
You will need to track the performance of the ML model through pilot runs before you deploy it in the desired application. Once the model is tested and integrated into the workflow, you will need to monitor the output to ensure that it is providing the expected results.
- Make Necessary Changes
Your ML model may need to be re-trained to align with new trends and patterns, so ensure that your model is up-to-date.
- Hire an Automation Partner
Identifying use cases, choosing GenAI tools, and training models can get overwhelming. Hence, it is a good idea to work with an automation partner like Auxiliobits which can make the process seamless. Automation partners will work with you to establish your automation goals and implement the best tools and technologies according to the needs of your business.
Conclusion
Generative AI is reinventing finance by automating processes, creating synthetic data, and providing valuable insights for making strategic decisions. Integration of GenAI in financial services can unlock new opportunities, enhance efficiency, mitigate risks, and achieve better outcomes in a complex and dynamic financial landscape.
Generative AI has made a strong presence in the financial sector. With more user-friendly GenAI tools like Microsoft Copilot, Google Duet, and Amazon QuickSight on the horizon, the future of finance looks exciting!
If you are in the business of providing financial services to your clients, you will need advanced AI tools to stay competitive in a dynamic market. The professional team at Auxiliobits can provide your business with the best automation solutions for your business. Our fintech experts offer customized solutions for your brand. We help you identify the best tools and support you in their deployment and maintenance. Our experts work closely with your team to ensure a seamless digital transformation journey for your brand!
Download the eBook: Enabling CFO’s Office through HyperAutomation and Digital Transformation
To know more about how we empower CFOs with digital tools!