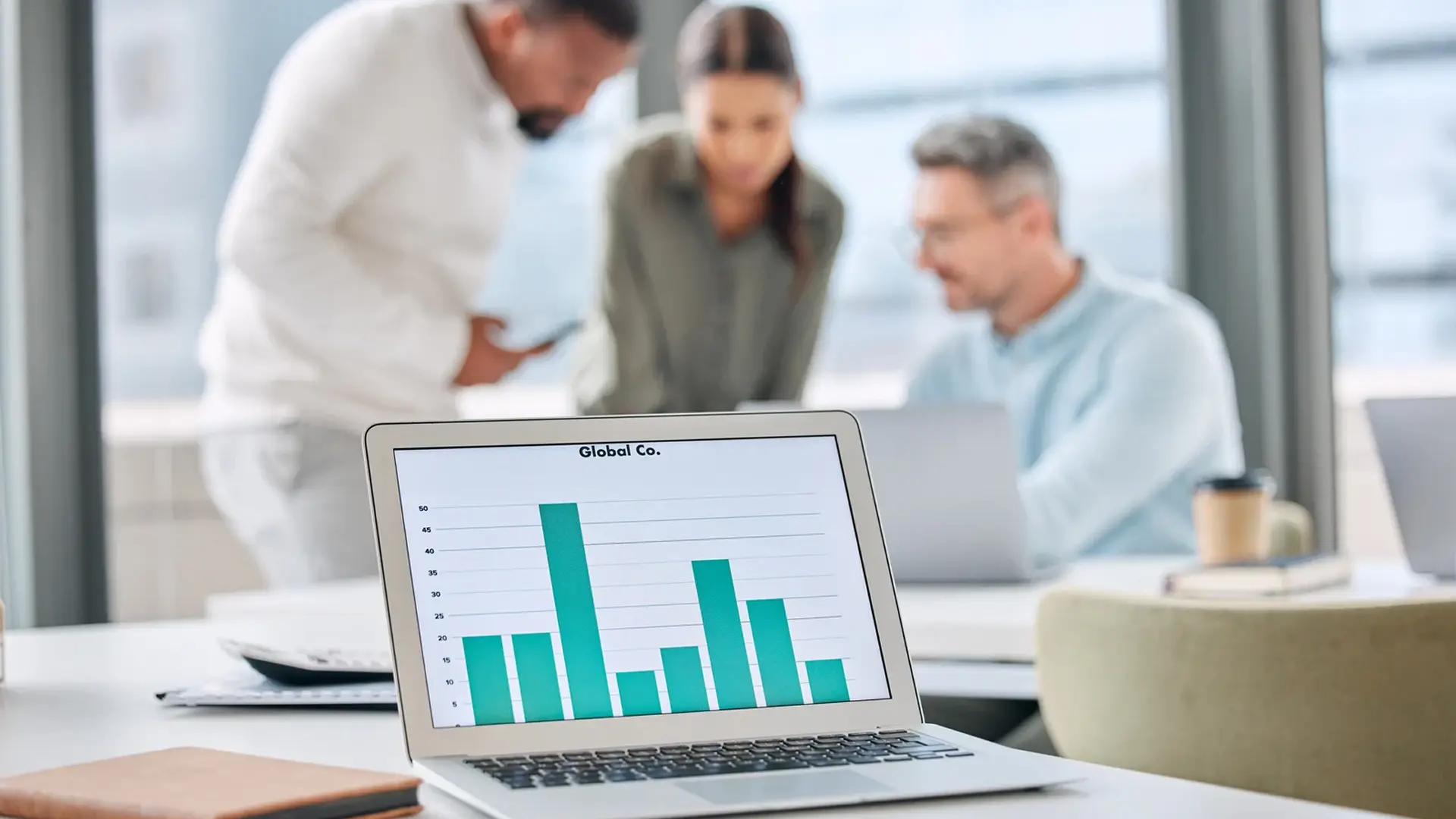
Key Takeaways
- It continuously learns from real-time data, adapting quickly to disruptions and trends, offering a more innovative, agile alternative to traditional static models.
- By analyzing news, social media, logistics, and economic indicators, Generative AI creates holistic forecasts that more accurately reflect real-world conditions than traditional tools.
- Generative AI simulates “what-if” scenarios, helping businesses prepare for uncertainties like supply shocks, demand spikes, or competitor moves before they happen.
- In data-scarce situations, AI generates synthetic data to model demand, train systems, and test strategies—ideal for new launches or rare disruptive events.
- AI co-pilots, self-healing chains, and eco-forecasting will improve planning, automate responses, and support environmentally responsible decisions across the supply network.
It is undeniable that supply chain forecasting is one of the most crucial elements to consider when wanting to run a successful business. This is because it helps firms identify all the products they need. It also helps them understand the quantity of the product and when it is a suitable time to get it. As a result, this helps firms know if they have enough inventory. Furthermore, it allows them to plan to ensure the operations do not hamper.
Gone are the days when companies used old data and statistical models to make predictions. Although these methods were beneficial, they did not work well, especially when the market situation changed. Today, a plethora of factors change markets. Global trends, disruptions in the supply chain, and customer choices affect demand. Therefore, traditional forecasting often struggles during such changes. This is where generative AI can function, ensuring businesses change their strategies to forecast and plan.
With the help of deep learning and advanced algorithms, generative AI can identify what customers like. Additionally, it understands everything trending on social media and the weather, making forecasts timely and accurate. Compared to old models, generative AI helps firms prepare themselves for changing situations when they don’t see them coming.
Also read: Leveraging Generative AI for Predictive Maintenance in Manufacturing Equipment.
The Limitations of Traditional Forecasting
Before we explore the advantages of Generative AI, it’s essential to understand the limitations of traditional forecasting methods. These older systems have served businesses for decades, but often fall short in today’s fast-paced, unpredictable market environment.
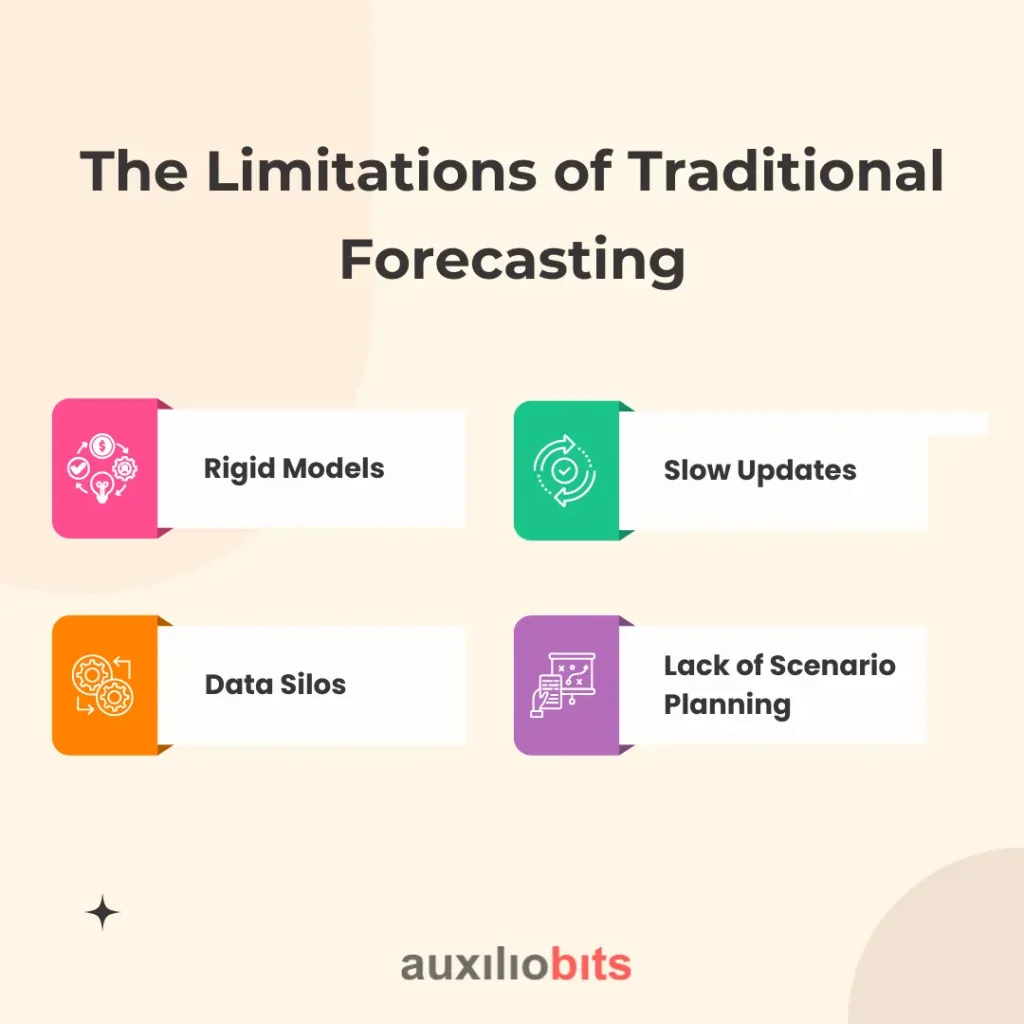
1. Rigid Models
Traditional forecasting methods usually rely on statistical models such as linear regression, moving averages, or ARIMA (Auto-Regressive Integrated Moving Average). These models are built to assume that future demand will follow historical patterns. While this works in stable environments, it becomes a significant problem when unexpected events occur. For instance, historical data was no longer a reliable predictor during the COVID-19 pandemic or the global semiconductor shortage. These rigid models lack the flexibility to adapt to sudden market shifts, making them ineffective in managing disruptions or anomalies.
2. Data Silos
Another major issue with traditional forecasting is its narrow focus on internal data, such as past sales records, inventory levels, or order history. While this data is valid, it only tells part of the story. External factors heavily influence modern supply chains, including geopolitical tensions, weather disruptions, economic indicators, and social media trends. Traditional tools are not equipped to integrate and process such diverse datasets. As a result, forecasts often miss critical signals, leading to inaccurate predictions and poor decision-making.
3. Slow Updates
Speed is critical in modern supply chain operations, yet traditional forecasting systems typically operate on fixed update cycles—weekly, bi-weekly, or monthly. This time lag means businesses are often reacting to outdated information. The market situation may have changed when new data is processed and forecasts are updated. This delay can result in overstocking, understocking, or missed sales opportunities. In contrast, real-time data processing is becoming increasingly essential for businesses to remain agile and responsive.
4. Lack of Scenario Planning
Traditional forecasting tools are also limited in their ability to model hypothetical or “what-if” scenarios. Supply chains are dynamic, and disruptions can happen without warning. However, older systems do not allow planners to test how different variables might impact outcomes. For example, they can’t easily answer questions like “What if a key supplier fails?” or “What if fuel prices double?” This inability to simulate alternate futures leaves businesses vulnerable and less prepared to deal with uncertainty.
These limitations make it clear that traditional forecasting alone is insufficient in today’s complex environment. A more adaptive, data-driven approach is needed—one that Generative AI can provide.
How Generative AI Solves These Challenges?
Generative AI is transforming the way businesses approach supply chain forecasting. Unlike traditional tools that rely on static models and historical data, Generative AI introduces flexibility, adaptability, and deeper insights. Here’s a closer look at how it overcomes the key limitations of traditional forecasting methods:
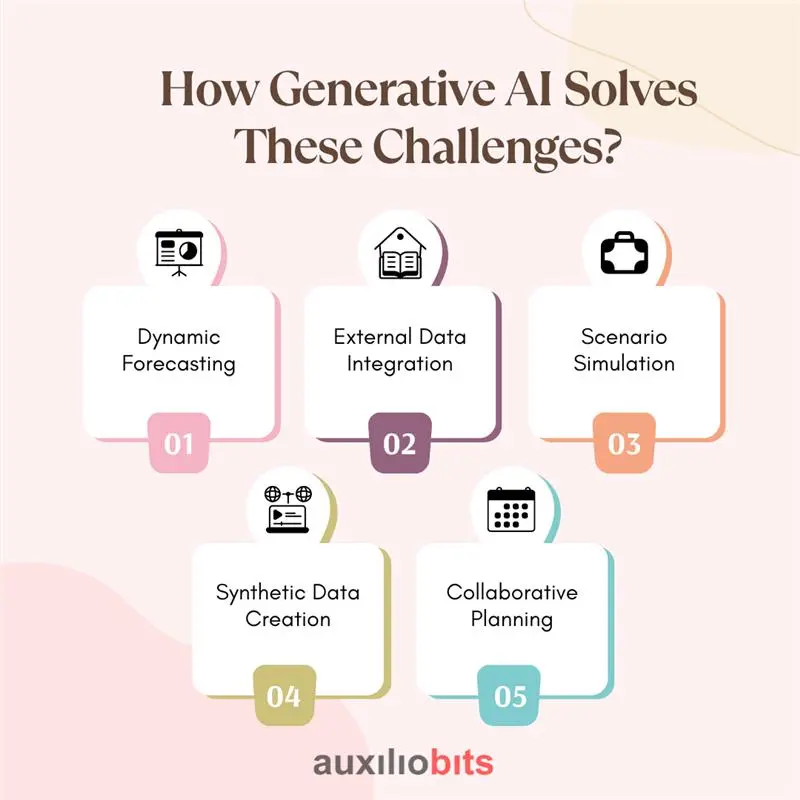
1. Dynamic Forecasting
One of the most powerful features of Generative AI is its ability to perform dynamic forecasting. These models are built using deep learning and neural networks that continuously learn from real-time data. Rather than waiting for a weekly or monthly update cycle, Generative AI can adjust forecasts instantly as new data comes in. For example, the AI can revise forecasts accordingly if a weather alert suggests a storm might affect shipping lanes or news headlines report political unrest in a supplier’s region. This constant learning loop ensures forecasts stay current, enabling faster, more informed decisions.
2. External Data Integration
Generative AI can handle large volumes of structured and unstructured data from internal and external sources. It can integrate insights from:
- Economic indicators like inflation rates, employment stats, or interest rates
- Global news feeds that report disruptions, policy changes, or health alerts.
- Customer reviews and social sentiment to detect shifts in demand or satisfaction
- Shipping and logistics data to track real-time delivery issues
- Competitor activities, including pricing changes, promotions, or product launches
Generative AI creates a much more comprehensive and context-aware forecast by processing this vast array of information. It doesn’t just look inward—it sees the complete picture of market dynamics.
3. Scenario Simulation
Generative AI excels at simulating a wide range of “what-if” scenarios. This is extremely valuable in today’s uncertain world. Supply chain managers can use the technology to model hypothetical situations, such as:
- What happens if a major supplier shuts down for two weeks?
- How will demand be affected if fuel prices increase by 25%?
- What’s the impact of a new competitor product on our forecast?
These simulations are not just surface-level—they incorporate multidimensional data and generate plausible future states. This helps companies identify risks early, explore contingencies, and plan mitigation strategies before problems occur.
4. Synthetic Data Creation
Generative AI can create synthetic data that mimics real-world patterns in cases with no historical data, such as new product launches or rare events. This is especially helpful for:
- Forecasting demand for brand-new products
- Testing supply chain resilience against low-frequency, high-impact disruptions
- Training AI models where historical examples are scarce
Synthetic data allows businesses to run simulations and train models without waiting months or years to gather real data. It fills critical gaps and improves forecasting accuracy.
5. Collaborative Planning
Generative AI can also serve as an intelligent assistant for supply chain teams. It doesn’t just provide forecasts—it offers recommendations and insights. For instance, the AI might flag a sudden spike in demand, suggest adjusting inventory levels, or recommend rerouting shipments. It also supports cross-functional collaboration by offering a shared, real-time view of supply chain performance. Logistics, sales, finance, and operations teams can work together more effectively, guided by AI-generated insights.
Generative AI redefines what supply chain forecasting can do by enabling smarter, faster, and more resilient decision-making.
Benefits of Generative AI in Supply Chain Forecasting
Benefit | Description |
Improved Accuracy | Forecasts are more precise, reducing waste and lost sales. |
Faster Response | Real-time insights allow businesses to act quickly. |
Flexibility | AI can adapt to unexpected events and new information. |
Better Collaboration | AI tools support teamwork by providing shared forecasts and suggestions. |
End-to-End Visibility | AI can connect data from every stage of the supply chain—from suppliers to customers. |
The Future of Supply Chain Forecasting
As Generative AI technology continues to grow and mature, it is set to revolutionize the supply chain further. The future of supply chain forecasting will be more automated, intelligent, and personalized than ever before. Here are some of the key trends we can expect:
1. Self-Healing Supply Chains
One of the most exciting possibilities is the rise of self-healing supply chains. In the future, AI systems will not just detect issues but automatically take steps to resolve them. For example, if the system notices that a shipment is delayed due to bad weather, it might automatically reroute other deliveries, notify customers, and reorder stock from a backup supplier. These AI-driven responses will happen in real time without waiting for human intervention. This level of automation reduces downtime, minimizes disruption, and improves customer satisfaction.
2. AI Co-Pilots for Planners
Forecasting tools of the future will be more interactive and user-friendly, acting like AI co-pilots for supply chain planners. Instead of relying on complex dashboards or spreadsheets, planners can have natural language conversations with the system. They might ask, “Why is the forecast for Product A increasing next week?” and receive a clear, data-backed explanation. These conversational interfaces will help teams make faster, more confident decisions while understanding the reasoning behind AI suggestions.
3. Sustainable Supply Chains
As sustainability becomes a key business priority, AI will significantly create greener, more responsible supply chains. Generative AI can recommend eco-friendly options for sourcing, manufacturing, and transportation. For instance, it might suggest using a regional supplier to reduce carbon emissions or identify routes with lower environmental impact. When generating forecasts, it can also factor in environmental regulations and corporate sustainability goals. This makes it easier for businesses to meet both operational and ethical targets.
4. Hyper-Personalized Forecasting
In the future, supply chain forecasts will become hyper-personalized, tailored to specific conditions and needs. Instead of one-size-fits-all estimates, businesses will get customized predictions for different regions, store locations, or customer types. A store in a tourist city during peak season will look very different from a small town’s forecast. This level of detail helps companies optimize inventory, reduce waste, and meet demand more precisely.
With these advancements, Generative AI is not just improving forecasting—it’s shaping the future of supply chain operations. The result will be more innovative, responsive, and sustainable supply networks.
Final Thoughts
Generative AI is a game-changer for supply chain forecasting. It offers dynamic, intelligent, and flexible predictions beyond traditional methods. While it requires investment and change, the benefits are clear: fewer surprises, better decision-making, and stronger supply chain resilience.
Businesses that embrace this technology today will be better prepared for tomorrow’s challenges—and better equipped to seize opportunities in an unpredictable world.