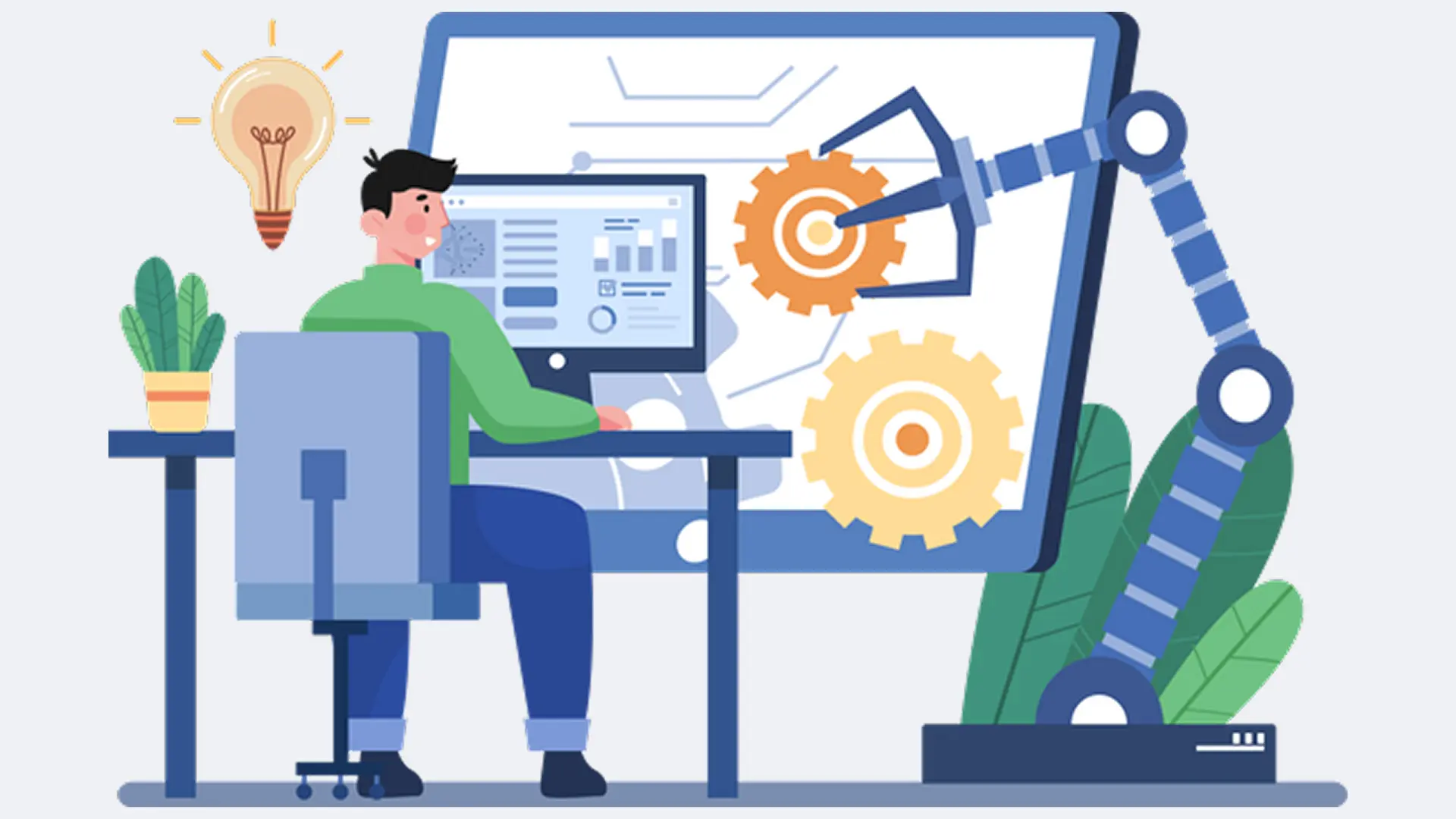
Key Takeaways
- It maps actual workflows using event logs, revealing bottlenecks, compliance gaps, and inefficiencies in financial services operations.
- AI models analyze historical data to forecast risks, delays, or customer churn, enabling more intelligent decision-making and process optimization.
- By combining process mining, AI/ML, and automation, financial institutions can act proactively, not reactively, across critical business functions.
- The integration ensures that workflows follow regulatory requirements and deliver fast, personalized service, enhancing trust and satisfaction in financial services.
- AI models and monitoring tools adapt over time, ensuring process efficiency and risk management improve as new data and patterns emerge.
The financial sector is under relentless pressure to provide better customer service. With so many responsibilities, they do not have sufficient time to focus on other tasks. Therefore, they have to enhance their accuracy, efficiency, and speed. Whether processing a loan application or identifying a customer’s loan history, financial settings should optimize operations to ensure that every customer is satisfied. However, all of this should be done fairly. This means that all essential rules should be followed while working.
Because financial firms cannot solve the challenges they come across, some of them have started utilizing predictive hyperautomation, powered by the benefits of process mining and artificial intelligence.
Process mining provides a data-driven lens to identify how business processes in financial firms run. This makes it easy for experts working in financial institutions to monitor compliance issues and inefficiencies. Therefore, they can obtain control over workflows and transparency. However, finding out what is happening is not similar to identifying everything that will happen in the future. This is where artificial intelligence comes into play.
Financial firms can operate better by integrating artificial intelligence with process mining. AI models will allow us to analyze historical patterns, identify early indications of inefficiency, and suggest corrective actions before customers are impacted. This change has allowed financial institutions to keep a check on fraud detection. In addition, they can also offer personalized customer service and more. Integrating process mining and artificial intelligence changes how business processes are created, executed, and improved.
Also explored is measuring ROI on Agentic Process Automation: Beyond Cost Reduction Metrics.
What Is Process Mining?
Imagine if your organization could see how every task is performed, not just how it’s documented in a manual or intended by design. That’s the power of process mining. It’s a technology that reveals how business processes truly unfold in real life, based on actual data from your IT systems.
Let’s say someone in your company is processing a mortgage application. They might go through various steps in a customer relationship management system, email platform, document management tool, etc. Every click, action, and update they make in these systems leaves behind a digital footprint, like a breadcrumb trail.
Process mining tools collect digital footprints (event logs) from systems like ERP (Enterprise Resource Planning), CRM, and core banking platforms. They then stitch this data together to reconstruct a visual representation of the entire process from beginning to end. It’s like building a map of your operations that shows not the ideal route but the actual path your teams follow daily.
With this map in hand, companies can answer key questions:
- Where are the delays and bottlenecks?
- Are employees skipping any critical steps?
- Are we meeting compliance standards?
- Which parts of the process vary across different teams or regions?
By uncovering the gaps between what should happen and what is happening, process mining gives businesses the insights they need to improve efficiency, reduce costs, and ensure compliance. It’s no longer about guesswork—it’s about data-driven decisions
What Is AI?
Artificial Intelligence (AI) is when machines are trained to simulate human intelligence. That means they can learn from data, identify patterns, make decisions, and improve over time. In financial services, AI is already making a significant impact.
Here are a few common examples:
- Chatbots that answer customer questions 24/7, without human involvement.
- Credit scoring models that evaluate loan applications in real time, using multiple data points.
- Fraud detection algorithms that flag suspicious transactions within seconds.
AI systems can process vast amounts of data far faster than humans and often more accurately. But AI is even more powerful when combined with real-world process data, precisely what process mining provides.
For instance, AI can detect and predict delays in a loan approval process based on historical trends and behaviors. Or imagine using AI to recommend the most efficient path through a complex insurance claims workflow, tailored to each case.
Bringing Them Together
When you combine process mining and AI, you move from understanding what’s happening in your business to predicting and optimizing it in real time. Process mining provides the transparency, while AI provides the intelligence. Together, they enable more intelligent decisions, faster responses, and more agile operations—especially critical in the fast-paced financial services industry.
What Is HyperAutomation?
HyperAutomation means automating everything that can be automated—including tasks, processes, decisions, and workflows—using multiple advanced technologies like:
- Robotic Process Automation
- Artificial Intelligence and Machine Learning
- Process Mining
- Low-code/no-code platforms
It goes beyond traditional automation by including decision-making and end-to-end orchestration.
Now Add Predictive Power: What Is Predictive HyperAutomation?
When you combine
- Process Mining (understanding how things work)
- AI/ML (learning patterns and predicting outcomes)
- Automation (doing things automatically)
You get Predictive HyperAutomation.
This means:
- Not only can you automate tasks, but
- You can predict problems before they occur, and
- Make intelligent decisions on the fly.
This is especially useful in financial services, where small mistakes can have enormous consequences.
Real-Life Example: Predicting Loan Approval Bottlenecks
Let’s say a bank has a loan approval process that’s partly manual and partly automated. Process mining reveals that 30% of loan applications are delayed due to missing documents.
By using AI on historical data, the bank can:
- Predict which applications are likely to be incomplete.
- Automatically send reminders or request documents upfront.
- Route complete applications through a fast-track workflow.
Result?
- Faster approvals.
- Happier customers.
- Lower costs.
Key Benefits of Combining AI and Process Mining in Financial Services
Combining Artificial Intelligence (AI) and process mining transforms financial services by unlocking new levels of efficiency, accuracy, and customer satisfaction. Here’s a detailed look at the key benefits this powerful duo brings to the industry:
1. Faster Processes
Speed is crucial in financial services. Delays can frustrate customers and risk losing business, whether approving a loan, processing a payment, or handling an insurance claim. Using process mining, organizations clearly understand where delays and bottlenecks occur within their workflows. For example, process mining can reveal that loan applications are stuck waiting for manual approvals or that claims processing slows down during specific steps.
Once these delays are identified, AI can help fix them proactively. AI-powered automation can accelerate data validation, document verification, and approvals. Moreover, AI algorithms can predict which applications or claims will likely get delayed, allowing staff to intervene early. This combination results in faster turnaround times, keeping customers satisfied and improving the company’s competitive edge.
2. Better Compliance
Compliance is non-negotiable in financial services, where regulations govern everything from anti-money laundering to customer data privacy. Process mining plays a vital role by continuously monitoring processes against compliance rules. It flags instances where procedures deviate from legal or regulatory requirements, such as missing mandatory checks or unauthorized access.
AI enhances this by monitoring data streams in real time for ongoing risks. For example, AI can detect unusual transaction patterns or inconsistent user behaviors that might indicate fraud or non-compliance. This allows compliance teams to respond quickly, reducing the risk of fines, legal issues, or reputational damage. Process mining and AI create a robust framework to ensure financial institutions stay on the right side of regulations.
3. Improved Customer Experience
Customers today expect fast, personalized, and hassle-free service. AI leverages customer data to predict each need—a loan offer, insurance coverage, or investment advice. AI-powered automation ensures these needs are met quickly by streamlining the underlying processes.
Meanwhile, process mining ensures the consistent and error-free delivery of these services. It tracks every step of the customer journey, verifying that workflows are executed as designed and identifying any points where mistakes or delays could occur. This combination speeds up service and builds trust by minimizing errors and improving transparency, leading to higher customer satisfaction and loyalty.
4. Cost Savings
Financial institutions handle massive volumes of repetitive tasks, such as data entry, document processing, and transaction verification. Automating these tasks using AI significantly reduces labor costs. But cost savings go beyond just cutting staff hours—automation also minimizes errors that can lead to expensive penalties, rework, or customer churn.
Process mining identifies which parts of the process are most time-consuming or error-prone, helping prioritize automation efforts where they will have the most significant impact. By streamlining operations and eliminating inefficiencies, organizations can optimize resource use and reduce operational costs, freeing budget for innovation and growth.
5. Proactive Risk Management
Risk is inherent in financial services, from fraud and credit defaults to operational failures. By analyzing real-time data, AI can detect unusual patterns that may indicate emerging risks. For example, a sudden spike in insurance claims could signal fraud or a systemic issue requiring investigation.
Process mining supports this by providing a clear view of process flows, helping risk teams understand where and why deviations happen. Together, AI and process mining enable financial institutions to move from reacting to risks after they occur to proactively identifying and mitigating them before they escalate. This proactive approach protects the business and its customers, strengthening overall resilience.
In summary, integrating AI with process mining empowers financial services companies to operate faster, safer, and cost-effectively while delivering a superior customer experience. This dynamic revolutionizes how finance firms manage complexity and compete in today’s fast-moving digital landscape.
How It All Works Together: The Step-by-Step Flow of Predictive HyperAutomation
Let’s break down the workflow into clear, actionable steps to understand how Process Mining and AI transform financial services. This step-by-step flow explains how data, insights, and automation combine to create a seamless, intelligent system.
Step 1: Data Collection
Every digital interaction within an organization leaves a trace. Whether it’s a customer applying for a loan through a CRM system, a transaction recorded in a core banking platform, an email communication, or even data maintained in spreadsheets, these systems continuously generate event logs. These logs are time-stamped records of each action taken within the system—who did what and when.
In this first step, all these logs from multiple sources are gathered into a centralized data repository. This comprehensive collection ensures no piece of the process puzzle is missing, giving a holistic view of how workflows operate across systems and departments.
Step 2: Process Mining
Once the data is collected, process mining tools analyze these logs to reconstruct the process flow. Unlike traditional methods that rely on documented workflows or assumptions, process mining reveals what truly happens on the ground. It identifies each step, its length, and how processes vary between cases.
This analysis helps uncover bottlenecks where tasks slow down, identify repeated or skipped steps, and detect deviations from compliance standards. For financial services, this means understanding precisely where loan approvals get delayed, where fraud detection checks might be bypassed, or where customer service processes falter.
Step 3: AI/ML Modeling
With a clear picture of past and current processes, the next step involves using historical data to build machine learning models. These AI models learn patterns from the data, such as what conditions typically lead to processing delays, fraud attempts, or customer drop-offs.
These models become predictive engines that anticipate future outcomes based on ongoing process data. For example, if a loan application shows signs that it’s likely to be delayed, the system can flag this early, allowing intervention before customers get frustrated.
Step 4: Decision Rules + Robotic Process Automation
Prediction alone isn’t enough; action is critical. In this step, organizations create automated decision rules and integrate RPA bots that can take immediate action based on AI insights.
- If the system predicts a delay, it can automatically alert a manager to expedite the case.
- The process can be halted to prevent losses if suspicious activity resembling fraud is detected.
- A retention offer can be triggered automatically if a customer shows signs of churn.
These automated responses ensure processes are monitored and dynamically optimized without constant human oversight.
Step 5: Continuous Monitoring and Improvement
The final step is an ongoing cycle. The system continuously monitors and learns new data, updating AI models to improve accuracy. It automatically detects new patterns and emerging risks, ensuring the organization adapts quickly to changing conditions.
This creates a self-improving, intelligent process ecosystem that evolves with the business, driving sustained operational excellence and superior customer outcomes.Together, these steps show how combining process mining and AI transforms raw data into actionable insights and automated decisions, enabling financial institutions to operate smarter, faster, and more confidently.
Conclusion
Predictive HyperAutomation is not just a buzzword—it’s a powerful combination of AI and process mining that enables financial services companies to work smarter, faster, and more accurately. By predicting problems and automating decisions, businesses can unlock new levels of efficiency and customer satisfaction.
If your financial institution wants to stay ahead in the digital race, now is the time to explore this game-changing approach. Start small, scale fast, and embrace the future of intelligent finance.