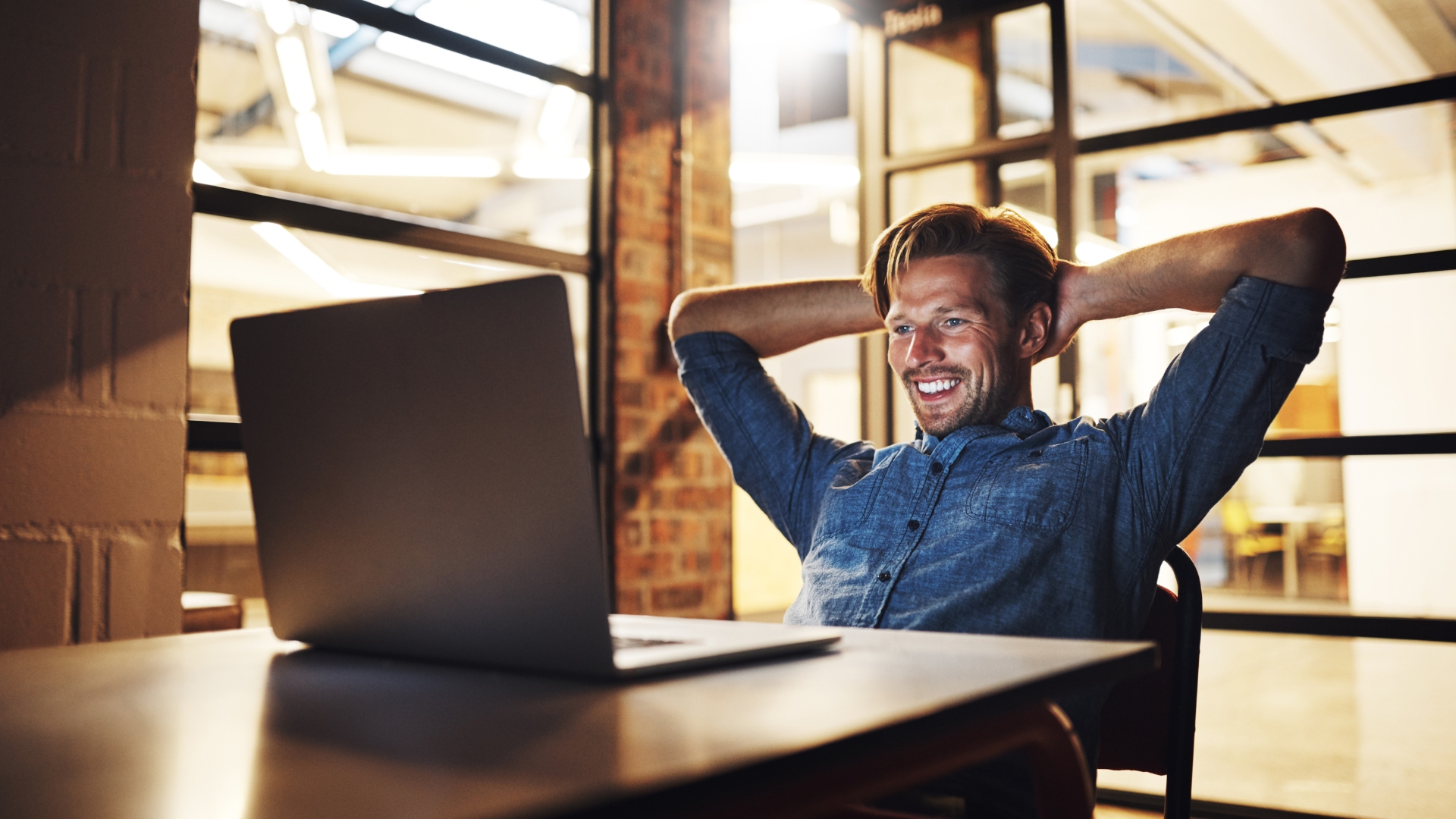
Key Takeaways
- Enterprises are moving beyond task-based automation to AI-driven autonomy, enabling intelligent decision-making and innovation with minimal human oversight.
- Combining AI with RPA enables businesses to automate complex workflows, learn from data, and reduce dependency on fixed rules and human intervention.
- AI agents now demonstrate strategic planning, contextual understanding, and independent decision-making, transforming operations across logistics, finance, and manufacturing sectors.
- A robust digital infrastructure, data governance, cloud platforms, and ethical frameworks are essential for responsibly deploying and scaling AI systems.
- Autonomous systems will not replace humans but enhance their potential, ushering in new roles focused on creativity, strategy, ethics, and system design.
Over the past few decades, AI has offered numerous perks to everyone. Businesses have scaled their operations while customers get recommendations on which makeup to purchase. Artificial intelligence has done wonders for everyone. However, over the past few years, it has transformed how enterprises perform their operations, innovate, and add value to the customers. What originated as simple automation has become a valuable solution that does not require human supervision. This progression marks a significant shift in digital transformation, where organizations move beyond efficiency gains to building truly autonomous enterprises.
Simple automation relies heavily on pre-established guidelines to perform tasks. Nevertheless, firms identified that simple automation did not work best for them as the capabilities were limited. Apart from this, simple automation faced problems when adapting to several environments. Henceforth, a new dimension was introduced, referred to as innovation autonomy. Systems made rapid decisions using predictive analysis, natural language processing, and machine learning technologies. Apart from this, they identified patterns and intercepted data. All of these capabilities allow firms to move beyond task execution.
Firms must invest in data governance to understand the shift from simple automation to innovation autonomy. Not only this, but they should also invest in partnership and ethical frameworks. One primary reason behind this is that to climb the ladder of success, it is essential to align AI initiatives with objectives that support innovation. Firms need to understand that the shift from automation to autonomy is not just an upgrade but a necessary shift in how firms operate. Scaling AI effectively is a paramount enabler of this transformation, closing the gap between automation and autonomous enterprise operations.
Also read: The Future of AI-Driven Digital Twins in Smart Factories.
Understanding Automation: The Foundation of Digital Transformation
Automation has long been a cornerstone of operational efficiency. It began with scripts, macros, and software tools to execute repetitive tasks quickly and accurately. These early automation efforts were timed to reduce human error, increase productivity, and cut costs.
In industries such as manufacturing, automation was driven by programmable logic controllers (PLCs) and robotic arms. In the business world, robotic process automation (RPA) emerged as a game changer, allowing enterprises to automate rule-based tasks across various departments, such as finance, HR, and customer service. However, these systems lacked cognitive capabilities and required significant human oversight. While traditional automation brought substantial benefits, it was inherently limited by its dependence on static rules and predefined workflows. Any variation in input or unexpected scenario could disrupt the process, necessitating human intervention. This paved the way for more intelligent solutions.
The Rise of Intelligent Automation: Combining AI and RPA
Integrating AI technologies, such as natural language processing, machine learning, and computer vision with RPA marked the beginning of intelligent automation. This new approach enabled systems to process unstructured data, learn from historical patterns, and adapt to changing conditions. For instance, intelligent document processing can extract, classify, and validate data from various document formats, significantly enhancing accuracy and efficiency. Chatbots powered by NLP can understand and respond to customer queries in real time, offering a more personalized and scalable customer experience.
Intelligent Automation allows organizations to handle more complex use cases that were previously beyond the scope of traditional RPA. It bridges the gap between deterministic rule-based automation and cognitive capabilities, enabling decision-making based on real-time data. However, while intelligent automation enhances capabilities and reduces manual intervention, it still requires human governance for exceptions, continuous training of AI models, and process orchestration. This necessity led to the emergence of the next phase in the evolution of AI: autonomy.
Moving Toward Autonomy: Agentic AI and Decision-Making Systems
Autonomy in AI represents a paradigm shift. Systems execute tasks, make independent decisions, adapt to new situations, and continuously learn with minimal human input. This is made possible through advancements in Agentic AI, where autonomous agents operate based on goals, environmental data, and feedback mechanisms.
These AI agents are capable of:
- Contextual understanding
- Continuous learning
- Strategic planning and goal-setting
- Collaboration with other agents and systems
For example, in supply chain management, autonomous AI can monitor global logistics data, predict potential disruptions, optimize delivery routes in real time, and automatically communicate with vendors or customers without waiting for human instructions.
This level of autonomy is underpinned by sophisticated algorithms, deep neural networks, reinforcement learning, and large language models (LLMs) that process vast amounts of data and derive actionable insights. The result is a significant leap in business agility, responsiveness, and resilience.
Enabling AI at Scale: Infrastructure, Data, and Governance
Achieving autonomy at scale requires a robust digital infrastructure. Enterprises must invest in scalable cloud platforms, data lakes, and AI model deployment pipelines. The democratization of AI tools through platforms like Azure, AWS, and Google Cloud has made it easier for businesses to train and deploy models with agility.
Data is the fuel for autonomy. High-quality, diverse, and well-governed data sets are essential to train accurate and unbiased models. Establishing data governance frameworks ensures compliance, security, and ethical AI usage.
Moreover, scaling AI demands change management, cross-functional collaboration, and workforce readiness. Employees must be trained to work alongside intelligent systems, and leadership must foster a culture that embraces innovation and continuous improvement.
Real-World Applications: From Assisted to Autonomous Operations
Organizations across industries are already reaping the benefits of AI at different maturity levels:
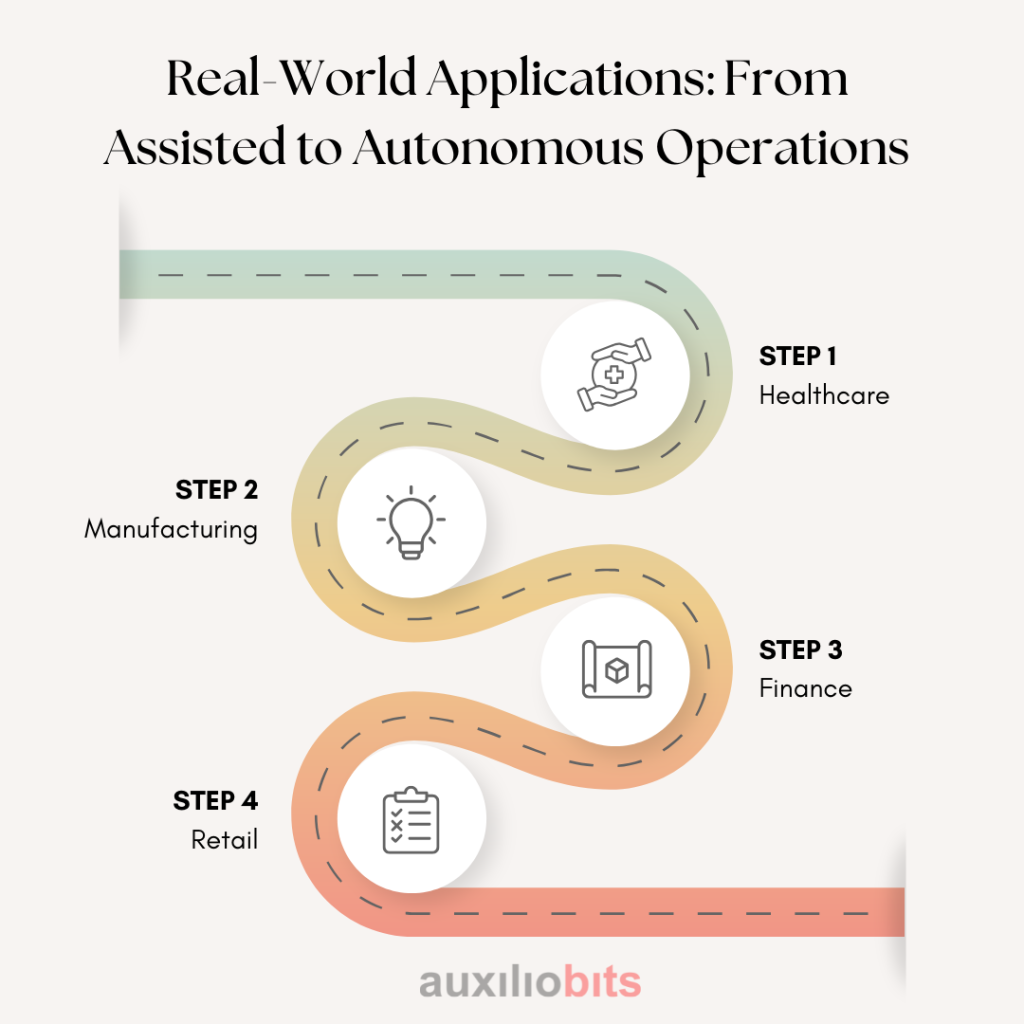
1. Healthcare
AI agents assist in diagnostics, monitor patient vitals, and predict disease outbreaks. Thoroughly autonomous systems can recommend treatment plans or manage hospital workflows.
2. Manufacturing
Smart factories use AI to monitor equipment, detect anomalies, and initiate preventive maintenance autonomously.
3. Finance
Autonomous trading systems analyze market trends and execute trades. AI agents detect fraud in real time and ensure regulatory compliance.
4. Retail
Autonomous AI systems increasingly manage personalized shopping assistants, inventory optimization, and dynamic pricing engines.
These examples demonstrate the progression from assisted operations to autonomous decision-making, where AI is not just a tool but an active participant in business ecosystems.
Challenges and Ethical Considerations
As businesses move toward full autonomy, several challenges must be addressed:
1. Trust and Transparency
Autonomous systems must be explainable and transparent to build stakeholder trust.
2. Bias and Fairness
Ensuring AI decisions are fair, unbiased, and aligned with ethical standards is crucial.
3. Security and Privacy
Autonomous AI must operate within secure environments to protect sensitive data.
4. Regulatory Compliance
Organizations must stay updated with evolving AI regulations and compliance requirements.
Addressing these challenges requires collaboration between technologists, policymakers, and business leaders to establish ethical frameworks and industry standards.
The Future of Work: Human-AI Collaboration in Autonomous Enterprises
As AI continues its evolution, the nature of work is also transforming. The future will be defined by synergistic collaboration between humans and intelligent systems. Rather than replacing jobs, autonomy will augment human capabilities, enabling employees to focus on creative, strategic, and relationship-centric tasks.
Roles such as AI trainers, ethical AI auditors, and automation architects will become integral to autonomous enterprises. Continuous upskilling and a focus on human-centric design will ensure that technology serves people, not the other way around.
Conclusion
The journey from automation to full autonomy is a technological shift and a strategic imperative for future-ready organizations. By utilizing AI at scale, enterprises can achieve unprecedented efficiency, innovation, and customer satisfaction.
While the road to autonomy presents challenges, the potential rewards outweigh the risks. Organizations that proactively adapt this transformation, invest in the right capabilities, and align technology with purpose will lead tomorrow’s autonomous economy. In essence, full autonomy is not the end of the road—it is the beginning of a smarter, more adaptive, and more human-centric future.