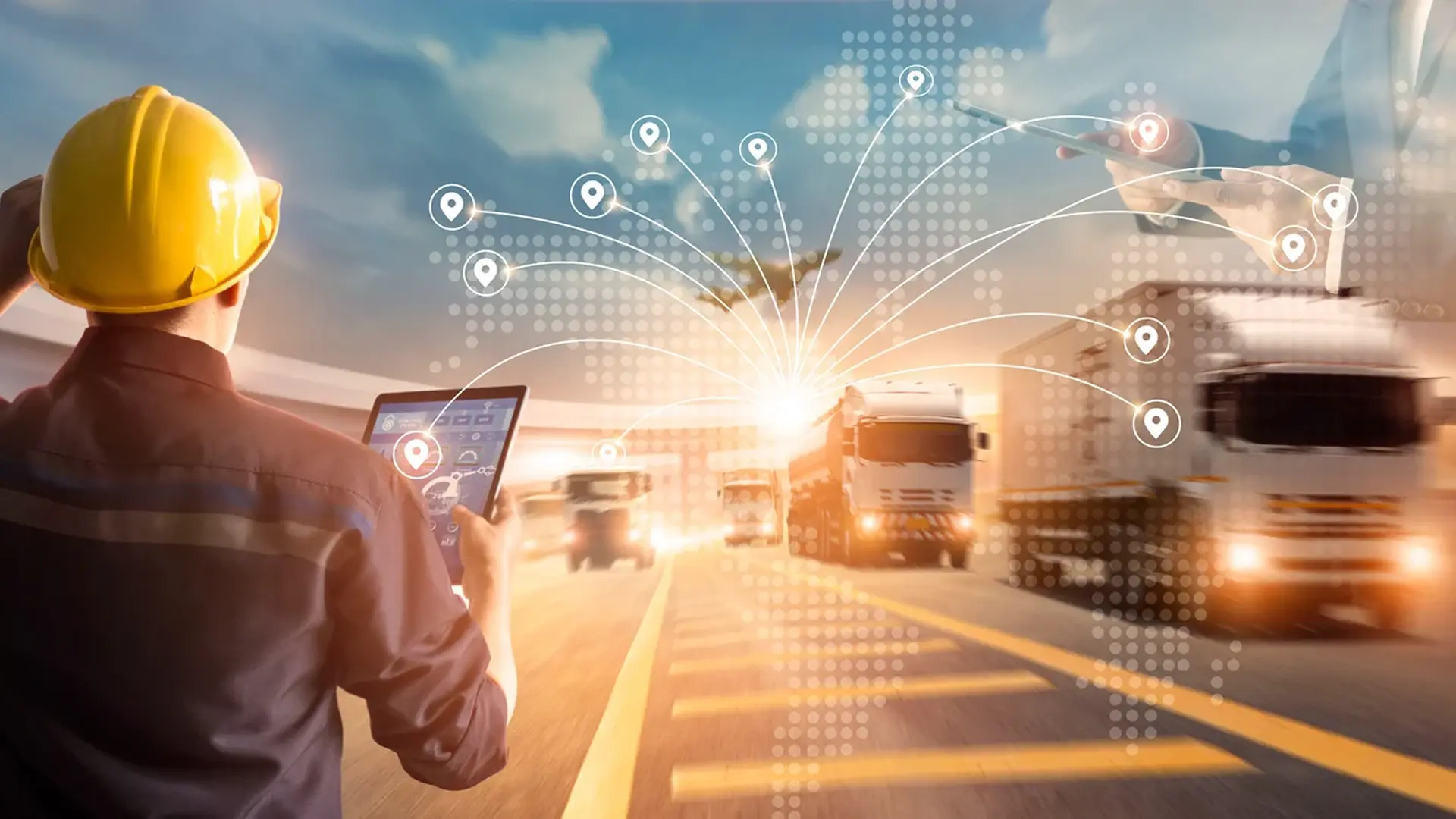
Key Takeaways
- AI-powered predictive maintenance enables logistics firms to avoid costly breakdowns and delays by identifying potential issues early, leveraging real-time data from vehicle sensors and intelligent machine learning algorithms.
- IoT sensor integration in delivery vehicles enables continuous data collection on vital parameters, such as tire pressure and engine temperature, providing the foundation for accurate predictive analytics and timely maintenance scheduling.
- Centralized data systems, combined with AI models, enhance decision-making by transforming raw sensor data into actionable insights, ensuring that vehicles are serviced only when truly necessary.
- AI enhances fleet safety and longevity by detecting anomalies early, reducing wear and tear, optimizing repair schedules, and minimizing the risk of on-road failures, thereby improving regulatory compliance.
- Predictive maintenance reduces operational costs by minimizing unplanned downtime, optimizing resource allocation, and eliminating unnecessary part replacements, thereby enhancing customer satisfaction and driving business scalability.
The logistics settings function is crucial in an environment where timely deliveries are essential. If there is any delay, the firms might end up facing unwanted consequences. The performance of trucks, vans, and delivery vehicles has a direct impact on operational success. If there are sudden maintenance issues or breakdowns, there is a high chance of deliveries being delayed. This way, not only will the business be hampered, but the customers will also lose trust. Henceforth, to mitigate such challenges, predictive maintenance is a suitable approach. However, when combined with artificial intelligence, predictive maintenance becomes even more effective. Sensors embedded in delivery vehicles gather all the details, including tire pressure, brake performance, and engine temperature. AI algorithms process all this data to identify anomalies, breakdowns, and other issues, allowing logistics firms to schedule maintenance efficiently without wasting time.
Implementing AI-driven predictive maintenance comes with a structured approach. Firstly, firms should ensure that all their delivery vehicles are equipped with IoT sensors and connect these to a centralized data platform. Secondly, all the records and operational data are fed into machine learning (ML) models that help identify failures. These models enable firms to refine their data over time. The final step is to integrate predictive details into maintenance planning, ensuring timely deliveries.
The advantages of AI for predictive fleet maintenance in logistics are numerous. From reduced vehicle downtime to reliable delivery and enhanced customer service, the benefits are multiple. Hence, predictive maintenance should not be taken lightly if you are a business that wants to grow without facing any hurdles.
Also read: Why Should Small Businesses Consider Robotic Process Automation Services?
What is Predictive Maintenance?
Predictive maintenance is an advanced strategy that helps in monitoring the current condition and performance of equipment. One of the primary objectives is to determine if any equipment in the setting is not functioning correctly. The second goal is to identify if any necessary equipment is missing in the setting. This way, logistics firms can equip themselves and prepare for any potential failure.
Preventive maintenance follows a set timetable. This involves scheduling equipment maintenance, checking for potential failures, and reducing the likelihood of failures. Logistics firms are always prepared with a solution, as predictive maintenance utilizes technologies such as data analytics and the Internet of Things (IoT). The equipment is equipped with sensors that collect real-time data on various parameters, including temperature, vibration, pressure, oil levels, noise, and other relevant factors. This data is continuously analyzed using machine learning algorithms or statistical models to detect patterns and anomalies that indicate potential issues.
By acting only when data indicates a potential failure, companies can significantly reduce maintenance costs, improve asset reliability, and extend equipment life. In industries such as manufacturing, transportation, logistics, and energy, predictive maintenance plays a crucial role in ensuring operational efficiency and reducing downtime.
Role of AI in Predictive Maintenance
Artificial Intelligence (AI) significantly enhances the capabilities of predictive maintenance by turning raw data into actionable insights. In traditional maintenance setups, identifying potential equipment failures often relies on manual inspections or fixed schedules. AI transforms this process by enabling systems to learn from vast amounts of real-time and historical data, allowing for more accurate and timely predictions of equipment failures, particularly in dynamic environments such as fleet management.
AI-powered predictive maintenance begins with data collection through Internet of Things (IoT) sensors installed on vehicles. These sensors continuously monitor parameters such as engine temperature, oil pressure, vibration levels, fuel efficiency, and brake performance. The data is then transmitted to a centralized platform, where it is processed and analyzed using AI techniques, such as machine learning and advanced data analytics.
Machine Learning algorithms are trained on historical maintenance and operational data to recognize patterns associated with component wear and failure. Over time, these models become more accurate, identifying subtle anomalies that human analysis might miss. For example, a machine learning model could detect an unusual vibration pattern in a truck’s engine and predict a potential failure weeks before it occurs. AI also supports decision-making by prioritizing alerts based on risk levels and recommending optimal maintenance schedules. This enables fleet managers to intervene before breakdowns occur, reducing downtime, repair costs, and service disruptions.
Moreover, AI can continuously refine its predictions as more data is collected, making the system more innovative and more responsive over time. This adaptability is crucial for logistics operations where conditions vary widely based on routes, weather, and usage intensity.
Key Components of an AI Strategy for Predictive Maintenance
To successfully implement AI-driven predictive maintenance in logistics, the following components should be in place:
1. Data Collection Infrastructure
The first step is to install IoT sensors and telematics devices in fleet vehicles. These devices collect data on:
- Engine performance
- Fuel consumption
- Tire pressure
- Brake system status
- Battery voltage
- Temperature and vibration levels
2. Centralized Data Management System
All collected data should be stored in a secure and centralized system. A cloud-based platform is ideal, as it allows easy access, integration, and scalability. Data cleaning and standardization are also essential to ensure accuracy.
3. AI Model Development
Using machine learning algorithms, AI models can be trained to identify normal behavior and detect early warning signs of failure. The models learn from historical data and improve over time.
Key AI techniques used include:
- Regression Analysis – for predicting the remaining useful life of components
- Classification Models – for identifying failure types
- Anomaly Detection – for finding unusual behavior in vehicle performance
4. Predictive Alerts and Reporting
Once the AI model is trained, it should be connected to a dashboard or alert system. Maintenance teams should receive real-time notifications about predicted issues. The system should also generate reports on fleet health and recommended actions.
5. Integration with Maintenance Operations
The AI system should be linked to the existing fleet management or maintenance scheduling system. This enables the automatic creation of tasks, ordering of parts, and assignment of technicians based on predictions.
6. Continuous Monitoring and Improvement
AI models must be regularly updated with new data to maintain accuracy. Feedback from maintenance outcomes should also be used to improve the system. This ensures that predictions remain reliable over time.
Benefits of AI-Driven Predictive Maintenance in Logistics
AI-driven predictive maintenance offers a range of powerful benefits for logistics companies, enabling them to enhance efficiency, reduce costs, and improve service quality. By using real-time data and intelligent algorithms, logistics providers can maintain their fleets more strategically and proactively.
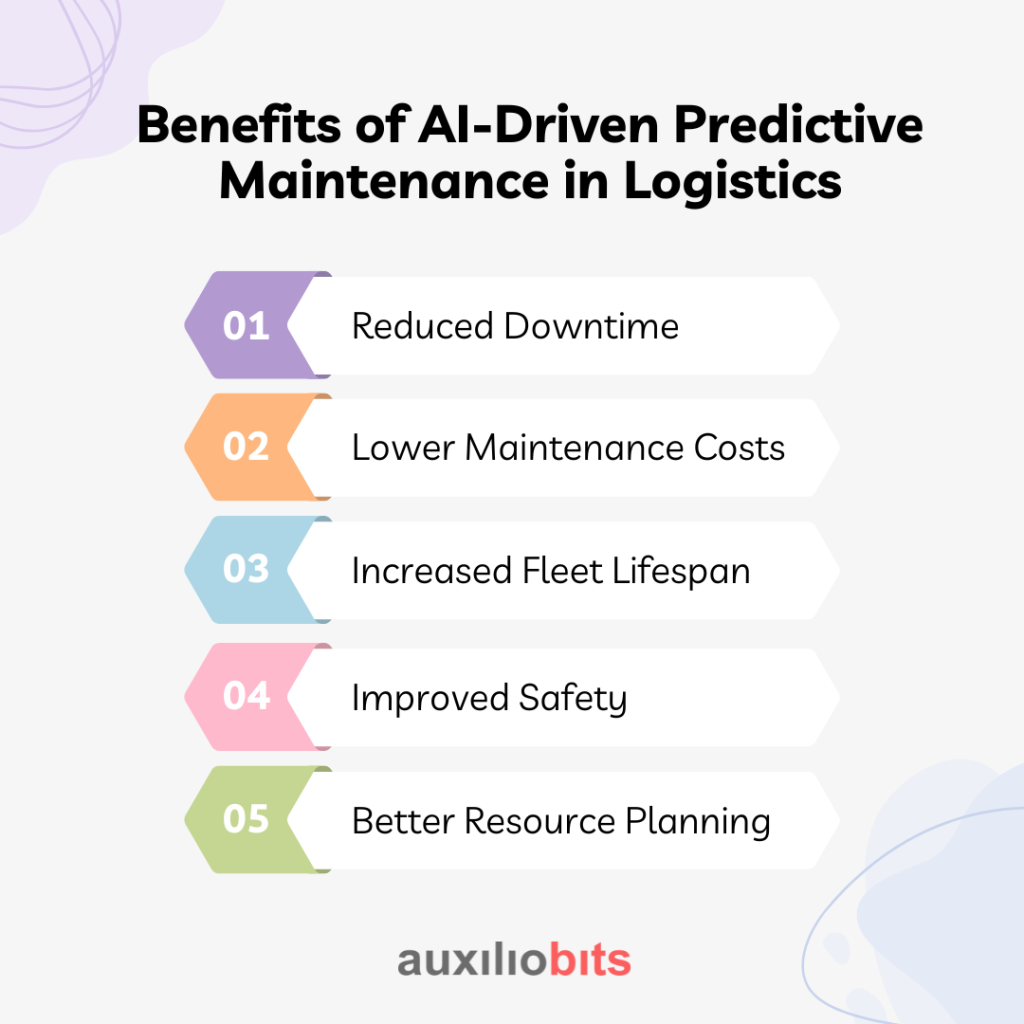
1. Reduced Downtime
ne of the most significant advantages is the reduction in vehicle downtime. With predictive maintenance, potential issues are identified and addressed before they escalate into serious failures. This ensures that vehicles remain operational and delivery schedules stay on track, ultimately leading to increased customer satisfaction and fewer service disruptions.
2. Lower Maintenance Costs
AI systems can pinpoint the exact components that require attention, eliminating the guesswork often involved in traditional maintenance. This precision helps avoid unnecessary repairs and reduces the cost of spare parts and labor. Instead of replacing parts on a fixed schedule, they are serviced only when needed, resulting in significant cost savings over time.
3. Increased Fleet Lifespan
Timely maintenance ensures that fleet vehicles operate at optimal performance levels. By preventing excessive wear and damage, the overall lifespan of the fleet is extended. This reduces the frequency of vehicle replacements, further lowering capital expenditures.
4. Improved Safety
By detecting potential failures early, AI-driven predictive maintenance minimizes the risk of breakdowns on the road. This enhances safety for drivers, passengers, and the general public while also helping companies comply with vehicle safety regulations and standards.
5. Better Resource Planning
Predictive insights allow fleet managers to plan maintenance activities more efficiently. Maintenance can be scheduled during off-peak hours, allowing for more effective management of technician availability, spare parts, and replacement vehicles, which ensures smooth operations.
Conclusion
Predictive maintenance using AI is a valuable solution for logistics companies seeking to enhance fleet reliability, reduce costs, and improve service quality. By following a structured implementation strategy, businesses can make more informed decisions, ensure timely deliveries, and establish a stronger market reputation.
AI does not replace human expertise; instead, it supports it by providing deeper insights and more innovative tools. With the right approach, predictive maintenance can transform fleet management into a more efficient and proactive process.