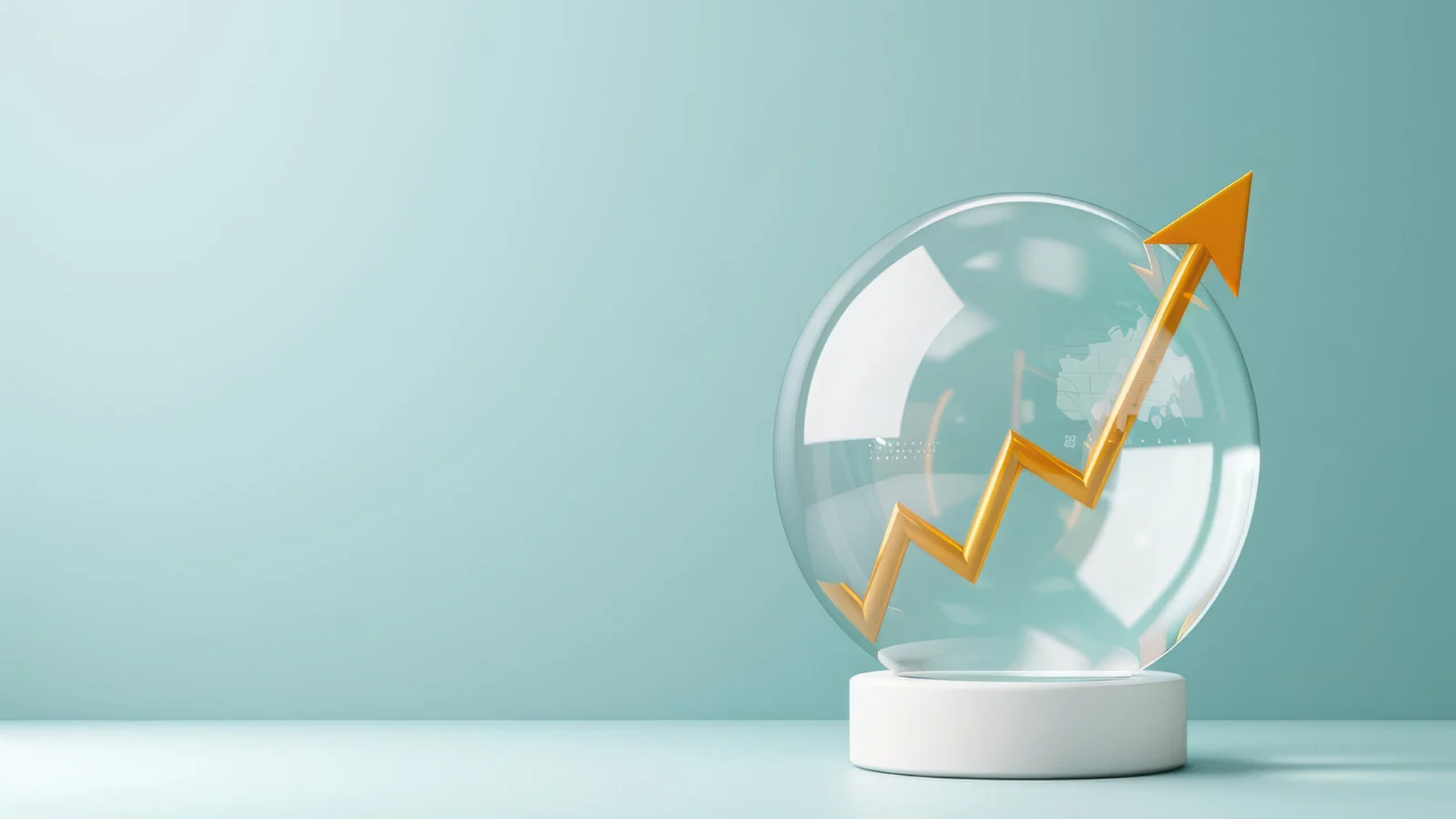
Key Takeaways
- AI enables real-time analysis of diverse data sources, enhancing forecasting accuracy by identifying trends, learning from behavior shifts, and quickly responding to market fluctuations.
- Conventional methods relying on historical data fall short during market volatility, failing to accommodate sudden changes in consumer behavior, external influences, and unstructured data.
- ML models evolve with new data, recognizing hidden patterns and adjusting forecasts dynamically—essential for volatile sectors needing agile and responsive planning.
- Unlike traditional methods, AI incorporates critical external drivers, such as competitor pricing, economic shifts, or public sentiment, creating a more holistic and realistic picture of demand.
- AI simulates multiple outcomes under various conditions, enabling businesses to prepare contingency plans and transition from reactive to proactive strategies in response to unexpected disruptions.
Accurate demand forecasting is paramount. No sector should take it for granted because it allows them to gain access to several benefits. Firms can not only optimize inventory but also make sure that their customers have a great experience. Additionally, it ensures that operations are smooth, thereby reducing expenses. Nevertheless, market uncertainty may pose challenges. To overcome these challenges, many firms have adjusted their strategies and begun utilizing artificial intelligence. This is because AI helps them enhance the agility of their demand forecasting procedures. Artificial intelligence also considers real-time data and has no dependency on outdated information. Hence, firms can monitor sudden market changes and adjust their strategies accordingly.
Also read: Why Will AI Agents Replace Traditional Automation?
What Is Demand Forecasting?
Demand forecasting is the process of predicting future customer demand for a service or product. Once all the needs are identified, firms can make informed decisions. This way, there is better cash flow and customer experience. Firms get an idea of items that need to be restocked. Apart from this, they can also make new additions if required. This way, new customers will be on board, and firms will be able to earn better returns.
Traditional demand forecasting depends on seasonal patterns and historical sales data to identify future requirements. If the market conditions are stable, these methods meet business requirements. Sectors including manufacturing, healthcare, and logistics have implemented these methods to align supply with demand. This has also helped them avoid stockouts and overstock. Nevertheless, in the present time, traditional forecasting is not reliable. Changes in the market, caused by the pandemic, economic shifts, and geopolitical tensions, have led to sudden shifts in demand. These are some of the factors that have given rise to unpredictability that models struggle to manage. Hence, this is where artificial intelligence can be utilized to gain better results. AI not only improves the accuracy of demand forecasts but also improves responsiveness. It enables firms to anticipate changes in demand, allowing for swift actions to be taken. Additionally, AI can integrate external data to provide a comprehensive understanding of demand drivers.
Why Traditional Methods Fall Short?
Traditional demand forecasting methods primarily rely on historical sales data, time-series analysis, and rule-based statistical models. These techniques are built on the assumption that past trends, patterns, and behaviors will continue. While this assumption may hold in stable and predictable market conditions, it quickly breaks down when faced with volatility, disruption, or rapid change.
One of the most significant drawbacks of traditional forecasting is its inability to respond to sudden changes in consumer behavior. Today’s customers are influenced by a wide array of factors—ranging from viral social media trends to global events—that can shift preferences and demand overnight. Conventional models, grounded in historical averages, often fail to account for these abrupt fluctuations, resulting in inaccurate forecasts and poor decision-making.
Another critical limitation is that traditional models often ignore external variables such as weather patterns, geopolitical developments, competitor actions, economic changes, or public sentiment. These external factors can have a profound impact on demand but are typically not integrated into rule-based models. For example, a sudden storm might spike the demand for certain goods, or a trending social media post might drive unexpected interest in a product. Traditional tools cannot incorporate and interpret such real-time, unstructured data.
Additionally, traditional forecasting methods are slow to adapt to new patterns. Market conditions, consumer preferences, and supply chain dynamics can change rapidly; however, statistical models may take weeks or months to adjust, as they rely on fixed rules and lagging indicators. This lack of agility can result in missed opportunities or overproduction, leading to increased costs and reduced efficiency.
Moreover, these methods typically require significant manual effort and expert involvement. Data must be collected, cleaned, and analyzed by specialists, making the process time-consuming and labor-intensive. This also introduces the risk of human bias or error, further reducing forecast accuracy.
How AI Improves Demand Forecasting?
AI has revolutionized demand forecasting by enabling businesses to make more accurate, data-driven predictions in an ever-changing and unpredictable environment. Unlike traditional methods, which rely on historical data and static models, AI-based forecasting systems can process large and complex datasets in real time, incorporating a broader range of variables that influence consumer behavior. Here’s how AI enhances demand forecasting and makes it more accurate and actionable:
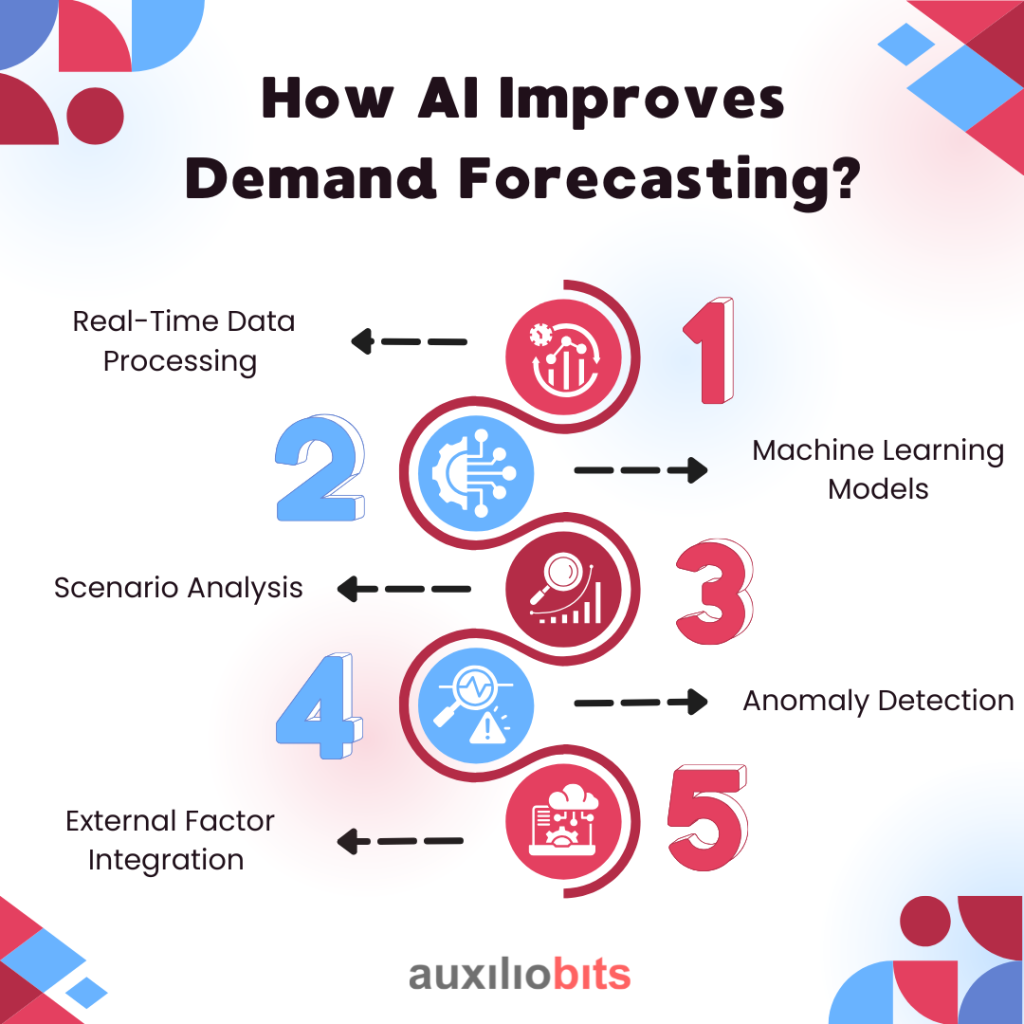
1. Real-Time Data Processing
ne of the most powerful features of AI in demand forecasting is its ability to process real-time data. Traditional methods often rely on historical data that might be outdated by the time decisions are made. AI tools, however, can continuously analyze live data from various sources, including sales platforms, web traffic, social media trends, and even weather systems. This capability enables AI to instantly adjust forecasts when new information becomes available, such as a sudden surge in online traffic or a shift in customer sentiment. The result is more timely and accurate predictions, allowing businesses to stay ahead of demand fluctuations and optimize their supply chain operations.
2. Machine Learning Models
Machine learning is at the core of AI-driven forecasting. Machine learning algorithms learn from past data, continuously improving their predictions by identifying hidden patterns and relationships that are often too complex for human analysts to detect. As new data is introduced, the model adapts and refines its forecasts, resulting in improved accuracy over time. This ability to learn and adjust makes machine learning models highly effective at identifying trends, even in highly dynamic environments. They can account for factors such as seasonality, shifts in consumer preferences, and subtle changes in buying behavior, providing businesses with a more nuanced understanding of future demand.
3. Scenario Analysis
AI can simulate multiple market scenarios to predict how demand might evolve under different conditions. By running “what-if” analyses, AI helps businesses anticipate best-case, worst-case, and most-likely scenarios, providing a range of potential outcomes. This allows organizations to be better prepared for unexpected events, such as supply chain disruptions or economic downturns, and develop more robust contingency plans. Scenario analysis is invaluable in decision-making, as it provides clarity on the potential impact of various factors and supports proactive adjustments to production, inventory, and marketing strategies.
4. Anomaly Detection
AI’s ability to detect anomalies in demand is another significant advantage. By analyzing historical patterns, AI can identify unusual changes in demand that may be caused by factors such as promotional campaigns, holidays, or unforeseen events, such as a viral social media post. This helps prevent false alarms and ensures that businesses can distinguish between normal fluctuations and exceptional circumstances. In turn, this leads to more reliable forecasts, reducing the likelihood of overstocking or understocking and minimizing operational disruptions.
5. External Factor Integration
AI’s ability to integrate external factors into its forecasting models further enhances its accuracy. Traditional models often overlook variables such as inflation, competitor pricing strategies, fuel costs, and public sentiment, all of which play a significant role in shaping demand. AI can analyze these external influences, adding another layer of insight to forecasts. For example, if a competitor lowers prices, AI can adjust demand predictions accordingly, or if there is an economic downturn, it can predict a decrease in consumer spending. Incorporating these external variables allows businesses to anticipate changes in demand that might otherwise be overlooked and adjust their strategies accordingly.
AI enhances demand forecasting by offering businesses a comprehensive, real-time, and adaptive approach to predicting customer demand. By leveraging machine learning, real-time data, scenario analysis, anomaly detection, and integration of external factors, companies can make more informed decisions, reduce risks, and optimize their operations for greater efficiency and profitability. AI-powered demand forecasting is no longer just a luxury—it’s a strategic necessity in today’s fast-paced, ever-changing business landscape.
Conclusion
AI-driven demand forecasting offers a more accurate and flexible approach to managing uncertainty in volatile markets. By combining multiple data sources, learning from patterns, and adapting to change, AI helps companies make informed decisions with confidence.
Adopting the proper techniques and models based on the industry and available data is key. With careful planning and proper execution, businesses can move from reactive to proactive planning and maintain a competitive edge, even in uncertain times.