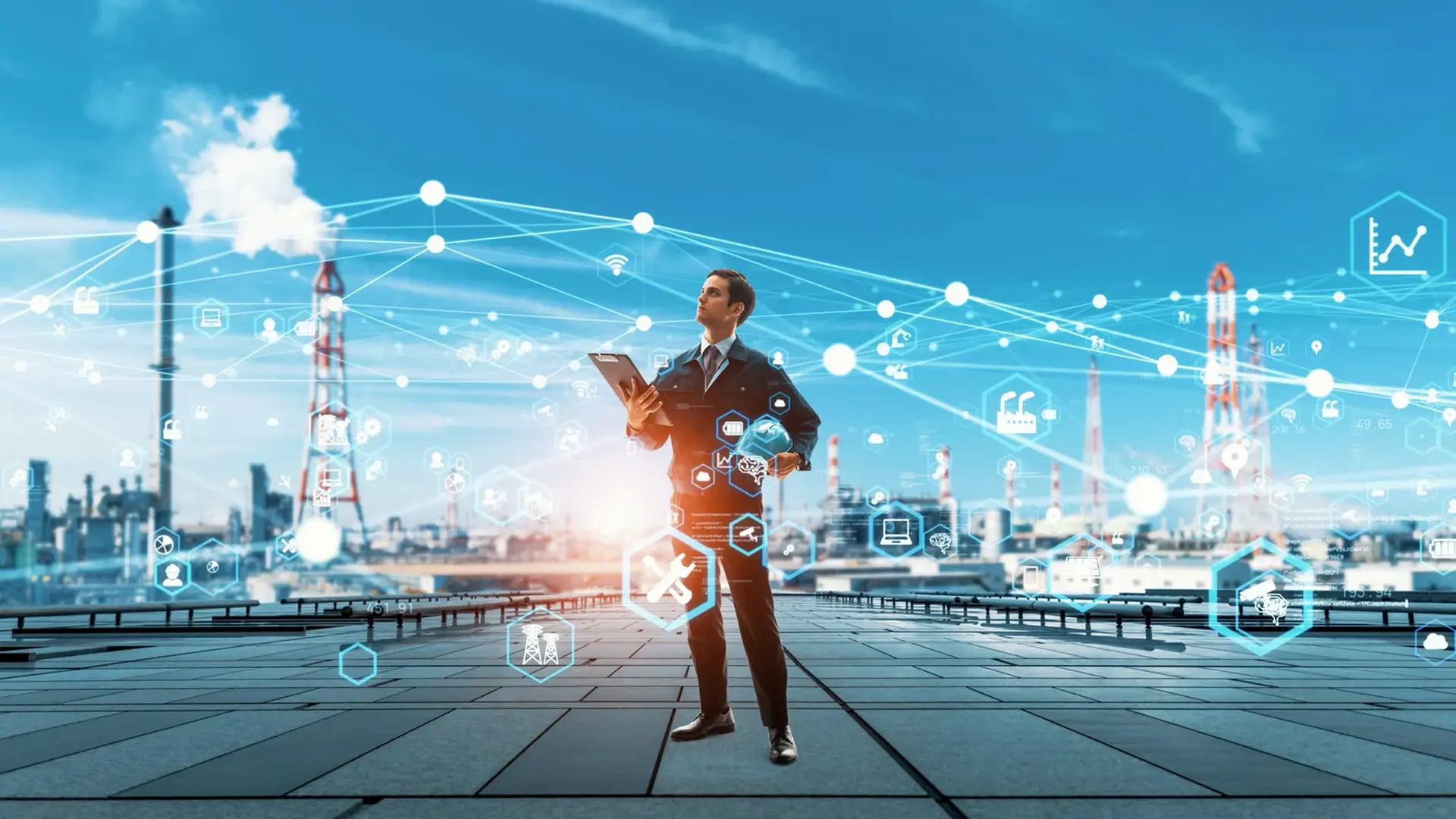
Key Takeaways
- Domain-specific AI agents enhance pharmaceutical processes by understanding industry-specific data, improving drug discovery, clinical trials, compliance, manufacturing, and supply chain operations.
- AI agents accelerate drug discovery by analyzing biomedical data and molecular structures, enabling the identification of potential compounds with greater precision, which significantly reduces development time and cost.
- In clinical trials, AI agents optimize participant selection, monitor patient data, and streamline trial management, reducing costs and improving safety and accuracy.
- AI ensures regulatory compliance by verifying documentation and monitoring manufacturing processes with IoT devices, thereby improving quality control, enhancing equipment performance, and minimizing risks.
- Training AI agents involves careful data collection, annotation, model selection, testing, and fine-tuning to ensure accuracy, reliability, and adherence to pharmaceutical standards.
Artificial intelligence has come a long way. It has left an indelible mark on several industries, healthcare and pharmaceuticals being among them. With market changes, numerous developments have occurred in various sectors. Nevertheless, one of the most innovative developments is the emergence of domain-specific AI agents. These agents are trained using data relevant to a specific domain. This includes documentation for drug development, regulatory guidelines, clinical trial records, and other appropriate materials. Specifically trained AI agents can now understand intricate terms, issues, and processes. This advanced comprehension enables them to perform tasks that previously required human intervention.
Now let’s talk about the pharmaceutical sector. This is where domain-specific AI agents are redefining numerous key procedures. For example, they help medical experts in drug discovery by recognizing potential compounds, predicting drug-target interactions, and analyzing molecular structures. In clinical trials, AI agents help optimize trial design and monitor patient data. Furthermore, in regulatory affairs, AI agents can smooth compliance checks, automate documentation, and ensure adherence to changing global standards.
If medical settings adopt domain-specific AI agents, they will gain access to numerous benefits. Decreasing operating expenses, improved information processing, faster decision-making, enhanced productivity, and a quicker time to market for new drugs are all benefits. Nevertheless, there are challenges, such as data privacy, but technologies like AI agents can help firms mitigate them if utilized effectively.
Also read: Designing Ethical Guardrails for Autonomous AI Agents in Financial Services
Key Areas of Pharmaceutical Process Automation
Pharmaceutical process automation consists of utilizing advanced technologies, including domain-specific AI agents. We have listed some of the key areas where domain-specific AI agents play an essential role below. Take a look:
1. Drug Discovery
Drug discovery is not an easy procedure. It comes with high costs and challenges. Therefore, years of experience and skills are required to get started. By examining amounts of scientific data, genetic details, chemical structures, and biomedical literature, domain-specific AI agents can help accelerate the entire process. Also, AI agents can identify trustworthy medication candidates by using learning algorithms. Hence, this improves the creativity and accuracy of medication development.
2. Clinical Trials
Do you know why clinical trials are of utmost importance? This is because they help in evaluating the efficiency and safety of new drugs. Nevertheless, they are at times plagued by increased expenses and delays. This is where AI agents help improve the efficiency of the procedure through various methods. Firstly, they help in analyzing the medical records of suitable participants, ensuring retention and effective patient recruitment. They also closely monitor patient data to ensure that professionals are prepared for any adverse situations that may arise.
3. Regulatory Compliance
Exploring the intricate regulatory requirements is a significant challenge that pharmaceutical firms face. AI agents help ensure compliance by verifying the accuracy of all regulatory documents. They also cross-check other essential materials for accuracy, alignment, and completeness, taking into account national and international guidelines.
Domain-specific AI agents are undoubtedly helpful in identifying anomalies that delay approvals. Additionally, they stay up-to-date and ensure that documentation and clinical data meet current regulatory standards, thereby reducing the risk of non-compliance.
4. Enables Manufacturing Automation
Automation driven by artificial intelligence enhances product quality and efficiency in the pharmaceutical industry. AI agents monitor every detail throughout the production process. From raw materials to finished products, everything is taken care of. Additionally, they collect and analyze data using IoT devices and sensors to identify anomalies and malfunctions in equipment. Moreover, they also help maintain strict quality control by identifying defects promptly without wasting time.
5. Supply Chain Management
A minimally invasive and efficient supply chain is crucial in ensuring quicker delivery of medicines and vaccines. Domain-specific AI agents improve supply chain performance by forecasting demand, managing inventory levels, and optimizing logistics. They utilize predictive analytics to anticipate potential disruptions, such as delays in raw material shipments or shifts in market demand, and automatically adjust their supply routes or sourcing strategies. By enhancing visibility and decision-making, these agents enable pharmaceutical companies to maintain a reliable supply chain that supports both patient needs and business objectives.
In summary, domain-specific AI agents are transforming pharmaceutical operations at every level. From innovation in drug discovery to ensuring regulatory compliance and supply chain efficiency, they enable smarter, faster, and more reliable processes, paving the way for a more agile and responsive pharmaceutical industry.
Training Domain-Specific AI Agents
Training domain-specific AI agents, particularly in complex and highly regulated industries like pharmaceuticals, is a meticulous and multi-stage process. These AI agents are not general-purpose systems—they must be deeply familiar with the intricacies of pharmaceutical language, data formats, workflows, and compliance standards. Building such an AI agent involves several key steps, each contributing to the agent’s ability to understand and make accurate decisions in real-world pharmaceutical scenarios.
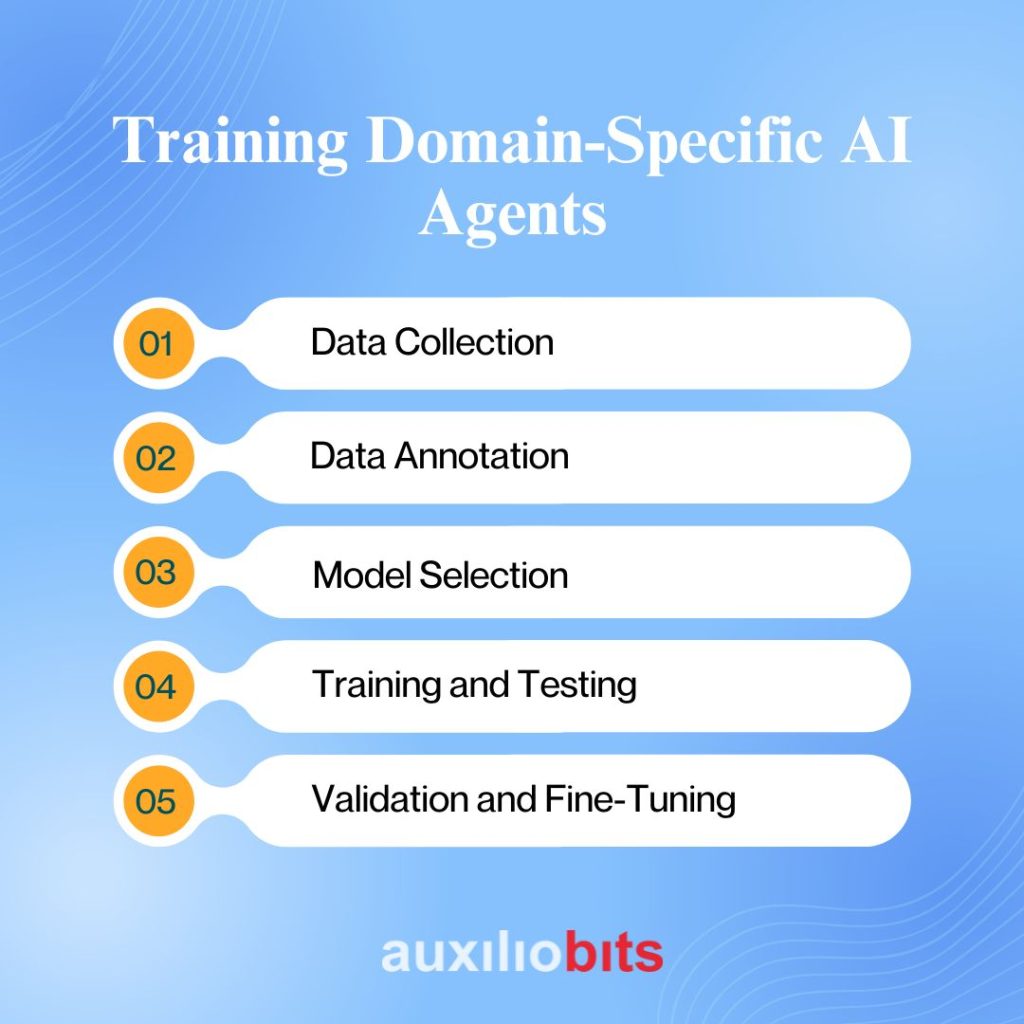
Step 1: Data Collection
The foundation of training any AI system is high-quality data. In the pharmaceutical domain, data are gathered from a wide range of credible sources, including peer-reviewed research papers, clinical trial databases (e.g., ClinicalTrials.gov), electronic health records (EHRs), drug manufacturing logs, adverse event reports, and regulatory documents from agencies such as the FDA or the European Medicines Agency (EMA). This data must be relevant to the specific tasks the AI agent is expected to perform. For example, if the agent is to assist with clinical trials, it needs access to past trial protocols, participant data, and outcome summaries. Ensuring that the data is diverse, accurate, and current is critical, as biased or outdated data can lead to poor model performance.
Step 2: Data Annotation
Raw data is often unstructured and meaningless to a machine without proper annotation. Data annotation involves labeling key elements within the datasets to help the AI understand their context. For instance, in a clinical trial document, entities like “drug name,” “dosage,” “symptom,” and “treatment outcome” may be marked accordingly. Specialized annotators—often domain experts or trained data scientists—utilize annotation tools to tag data accurately. In natural language processing (NLP) tasks, this may involve marking parts of speech, identifying named entities (such as diseases or medications), or labeling relationships between medical conditions and treatments. The quality of annotation directly affects how well the model learns the domain.
Step 3: Model Selection
With annotated data in place, the next step is to choose the appropriate machine learning (ML) model. The choice depends on the nature of the task—whether it’s classification, prediction, summarization, or natural language understanding. For tabular data, decision trees or gradient boosting machines might be used. For image recognition tasks, convolutional neural networks (CNNs) are a common choice. For functions involving text interpretation, NLP models such as BERT, BioBERT, or GPT-based models, which are fine-tuned for biomedical text, are highly effective. Model architecture and parameters are selected based on performance benchmarks and the complexity of the domain-specific tasks.
Step 4: Training and Testing
nce the model is selected, it is trained on the annotated dataset. During training, the model adjusts its internal parameters to minimize errors in its predictions or classifications. Training can take several iterations and involves continuous feedback loops. After training, the model is tested using a separate set of unseen data to evaluate its ability to generalize to new scenarios. This helps identify gaps in learning, overfitting, or underperformance in specific areas of the domain.
Step 5: Validation and Fine-Tuning
After initial testing, the AI agent undergoes validation, comparing its performance against real-world standards, expert expectations, and regulatory requirements. This stage involves rigorous fine-tuning, where model outputs are analyzed, and errors are corrected by retraining on targeted datasets or adjusting model parameters. The goal is to ensure the AI agent performs reliably and accurately across all tasks. In pharmaceutical applications, this step may also involve review by a domain expert and documentation to ensure compliance with industry standards and regulations.
By following these steps, domain-specific AI agents become valuable tools in pharmaceutical operations, capable of assisting in research, compliance, patient care, and other areas, all while adhering to stringent industry standards.
Conclusion
Domain-specific AI agents have the potential to transform the pharmaceutical industry. By automating complex and time-consuming processes, companies can become more efficient, reduce costs, and enhance the quality of their products. While there are challenges in training and implementing these agents, the benefits make them a valuable tool in the future of pharmaceutical process automation. With careful planning, secure data handling, and collaboration between humans and AI, the industry can achieve significant advancements in medicine and patient care.